Basics of Artificial Intelligence Algorithms
Artificial Intelligence (AI) is a fascinating field. It involves creating computer programs capable of learning and making decisions.
One of the key components of AI is algorithms. These are step-by-step procedures for solving problems.
Understanding the basics of AI algorithms is important for anyone interested in this rapidly growing field.
In this article, we will explore the fundamental principles of AI algorithms. We’ll also look at how they are used to power everything from recommendation systems to self-driving cars.
Whether you’re a beginner or an expert, this guide will provide valuable insights into the world of AI algorithms.
What are AI Algorithms?
AI algorithms are used in many industries. There are different types of AI learning: supervised, unsupervised, and reinforcement learning. Each type has a unique goal and method. This allows machines to learn from data and make predictions or decisions.
Machine learning and deep learning differ. Machine learning uses algorithms that can learn from and make predictions based on data, without being explicitly programmed. Deep learning uses algorithms to imitate the way the human brain processes data and creates patterns for decision making.
The most common AI algorithms are for predicting outcomes, grouping data, and classifying information. They include supervised learning, unsupervised learning, and reinforcement learning algorithms. These are vital in industries like healthcare, finance, and retail for automating decision-making, predicting outcomes, and managing work processes.
The rise of Large Language Models (LLMs) in AI algorithms has shown significant applications in various industries. It has also raised ethical considerations and challenges regarding bias and privacy. Responsible AI adoption is important to ensure the ethical development of AI algorithms.
Different Kinds of AI Learning
Supervised Learning: Being Taught with Answers
Supervised learning is about training a model on labeled data, using input data and corresponding correct output. This helps the model learn and make predictions with new data.
In unsupervised learning, the algorithm identifies patterns within unlabeled data without specific guidance.
Reinforcement learning trains a model to make sequences of decisions to achieve a goal through rewards and punishments.
AI algorithms used in supervised learning include linear regression, decision trees, support vector machines, and neural networks. For instance, a decision tree algorithm splits data based on specific features to create a tree-like structure for predicting the target variable.
Ensemble learning algorithms like gradient boosting and XGBoost improve the performance of supervised learning models by combining weak models into a stronger one. This reduces the risk of overfitting and improves generalization to new data.
Unsupervised Learning: Learning without Answers
Unsupervised learning is a type of artificial intelligence. It uses machine learning to find patterns in data without explicit target outputs. It’s different from supervised learning because it doesn’t need labeled outputs.
Reinforcement learning is where an AI learns by trial and error. It gets rewards or penalties for its actions. This is often used in situations where the AI must make a series of decisions.
Clustering algorithms in unsupervised learning group data points with similar traits. This helps recognize patterns and organize data without needing labeled data.
Reinforcement Learning: Learning through Trial and Error
Reinforcement learning is a type of machine learning. It enables AI algorithms to learn through trial and error. They make decisions and take actions to achieve specific goals.
Unlike supervised and unsupervised learning, reinforcement learning works by rewarding or penalizing the AI based on its actions and their outcomes.
This learning model is inspired by behaviorist psychology. It allows AI algorithms to continuously update their behavior based on the feedback received. This leads to improved decision-making and problem-solving abilities.
Real-world applications of reinforcement learning include:
- Autonomous vehicles learning to navigate with real-time data
- Robots learning to perform complex tasks
- Recommendation systems optimizing content based on user behavior
In business, reinforcement learning is commonly used to optimize supply chain management, improve resource allocation, and enhance financial trading strategies.
It offers significant potential for practical applications across various industries.
Comparing Learning Types: Supervised, Unsupervised, and Reinforcement
Supervised learning uses labeled data to train the algorithm. Unsupervised learning uses unlabeled data, and reinforcement learning utilizes trial and error with a system of rewards and punishments.
Supervised learning can make precise predictions and classify data accurately. However, it requires a large amount of labeled data and can be sensitive to overfitting.
Unsupervised learning allows for faster training since it doesn’t need labeled data, but it’s less accurate in predicting outcomes.
Reinforcement learning is advantageous in dynamic environments and can adapt to changes. However, it’s computationally expensive and has a slow learning process.
Supervised learning is commonly used in image recognition, language translation, and recommendation systems.
Unsupervised learning is often used in clustering, anomaly detection, and feature learning.
Reinforcement learning is used in robotics, game playing, and resource management.
Machine Learning vs. Deep Learning
Machine learning and deep learning are two different types of AI algorithms.
Machine learning uses algorithms to learn from data and make predictions. It’s simpler and requires less computational power.
Deep learning uses neural networks with hidden layers to automatically extract features from data. It’s more complex and suited for handling large volumes of complex data like image and speech recognition.
Machine learning is suitable for applications with limited resources or when transparency is important.
Deep learning is well-suited for solving complex problems.
Both approaches are important tools in AI, each suitable for different contexts.
Smart Algorithms: AI in Everyday Life
Big Brain AI: How Large Language Models Work
Large Language Models are a part of Big Brain AI. They use algorithms and neural networks to understand and create human language. These models are trained on large datasets of text to predict the likelihood of a word or phrase based on the context.
The main parts and processes involved in LLMs include training data, transformer architecture, attention mechanism, vocabularies, and positional encoding. LLMs can understand, create, and change human language, allowing for tasks like translation, summarization, and conversation with human-like responses.
They have potential applications in AI technology such as natural language understanding, chatbots, content generation, and sentiment analysis. LLMs can improve search engines, digital assistants, and speech recognition systems. However, they also raise concerns about privacy, bias, and ethical use of AI technology.
AI and Being Responsible: Making Sure AI is Safe and Fair
AI algorithms are designed to prioritize safety and fairness. This means eliminating biased data and outcomes. Transparent model training and evaluation can help eliminate bias and discrimination in AI learning. Responsible AI practices ensure that machine learning and deep learning models prioritize safety and fairness. This includes implementing strict ethical guidelines and continuously monitoring AI algorithms to prevent unintended biases.
Adhering to these practices ensures that AI decision-making aligns with safety and fairness principles.
Most Common AI Algorithms
Getting Predictions: Regression Algorithms
Linear regression is a basic type of regression algorithm in AI. It aims to model the relationship between a dependent variable and one or more independent variables. The algorithm uses linear regression to predict the value of the dependent variable based on the input of independent variables.
For example, when predicting house prices, linear regression can help determine the impact of factors such as location, size, and age of the property on its price.
Gradient descent is important for finding the best fit lines for making predictions with regression algorithms. It is an optimization algorithm that adjusts the parameters of the regression model to minimize the error between the predicted values and the actual values in the training data. By refining the model with gradient descent, the regression algorithm can identify the line that best fits the given data points, improving prediction accuracy.
Ensemble learning algorithms, including Gradient Boosting and XGBoost, help improve the accuracy of predictions in regression algorithms. These algorithms work by combining multiple individual models to form a more powerful, robust model. By leveraging the strengths of each individual model, ensemble learning algorithms reduce the impact of overfitting and underfitting, resulting in enhanced predictive performance for regression tasks.
For instance, in stock price prediction, ensemble learning can effectively capture the complex, nonlinear relationships between various market factors to yield more accurate forecasts.
Learning by Trend Lines: Linear Regression
Linear regression helps AI algorithms learn trend lines. It fits a best-fitting line to training data using gradient descent. This helps algorithms make predictions and minimize error between actual and predicted values.
For example, it can predict house prices based on features like square footage and number of bedrooms. By analyzing historical data, linear regression helps AI identify patterns and trends for decision-making and predictions. These insights contribute to accurate and efficient AI functioning, making it indispensable in artificial intelligence.
Fitting Best Lines: Gradient Descent
AI algorithms use gradient descent to find the best fitting lines in machine learning. This involves adjusting parameters to minimize a cost or loss function. In linear regression, gradient descent helps determine the best line fit by optimizing coefficients based on input data. While it’s good for large datasets and complex models, it can converge to suboptimal local minima instead of the global minimum.
Understanding gradient descent is crucial for training and optimizing machine learning models.
Choosing Groups: Clustering Algorithms
When choosing a clustering algorithm, it’s important to consider factors such as the dataset size, dimensionality, and the need for interpretability.
Clustering algorithms like K-Means help organize data into distinct groups. They do this by iteratively assigning data points to the nearest cluster centroid and updating centroids to minimize the within-cluster sum of squared distances.
K-Means has advantages in its simplicity and efficiency for large datasets. However, it has limitations in handling non-linear data distributions and determining the number of clusters.
On the other hand, Hierarchical clustering offers advantages in visualizing cluster dendrograms and not requiring the number of clusters as an input. But, it can be computationally expensive for large datasets.
Both clustering algorithms have different use cases and trade-offs. It’s important to carefully consider them based on the specific data and task at hand.
Making Groups: K-Means Clustering
K-Means Clustering is an AI algorithm that groups data points based on their similarities. The algorithm works by selecting random centroids, assigning data points to the nearest centroid, repositioning centroids, and reassigning data points. It converges when the centroids are stable and the groups are well-defined.
The steps for implementing K-Means Clustering involve selecting the number of clusters, initializing random centroids, assigning data points, recalculating centroids, and repeating until convergence.
In real-world scenarios, K-Means Clustering has potential applications in customer segmentation, pattern recognition, and anomaly detection. For instance, businesses can use it to segment customers, identify patterns in data, and detect anomalies in financial transactions. These applications showcase the practical value of K-Means Clustering in deriving insights from large datasets.
Figuring Out Categories: Classification Algorithms
Classification algorithms help in machine learning by sorting items into categories based on their features and attributes. This allows for organized and efficient data analysis. These algorithms learn from training datasets to identify patterns and assign input data to the correct classification. Common classification algorithms include logistic regression, decision trees, support vector machines, and k-nearest neighbors.
Each algorithm uses a different approach, such as using linear boundaries or proximity to other data points. Ensemble learning, which combines multiple models to improve predictive performance, minimizes errors and enhances accuracy. It creates a more robust and reliable classification system, delivering better results in various machine learning applications.
Decision Tree: Choosing by Asking Questions
The decision tree algorithm works by asking a series of questions and making classifications or predictions based on the answers.
In the category of classification algorithms, the decision tree algorithm sorts data into distinct categories based on specific features, playing a significant role.
When making predictions, the decision tree algorithm analyzes various factors and outcomes to make informed decisions based on identified patterns. These patterns can be used to predict future data based on learned associations.
The decision tree algorithm’s method of asking questions makes it a valuable tool in the field of artificial intelligence, where data sorting and predictive analysis are important components.
Logistic Regression: Yes or No Predictions
Logistic regression is an AI algorithm that predicts yes or no. It uses input data to calculate the probability of belonging to one of two categories. For instance, it can predict if an email is spam based on factors like the sender’s email address, keywords, and content.
Logistic regression is ideal for binary outcomes, like disease risk or customer churn. It’s commonly used when the dependent variable is categorical and has only two values, such as predicting if a customer will buy a product based on their purchase history.
However, logistic regression has limitations. It assumes a linear relationship between independent variables and the log-odds of the outcome. If the relationship isn’t linear, logistic regression may not be accurate. It’s also not suitable for complex data patterns and may struggle with too many independent variables.
Random Forest: Many Trees Making Decisions
The Random Forest algorithm uses a group of decision trees to make decisions. Each tree votes on the best outcome, and the most common decision is chosen. This method combines the power of multiple trees for a more accurate and robust result.
One advantage of Random Forest is its ability to handle large datasets with many features and variables. It’s also resistant to overfitting, meaning it can effectively adapt to new data, making it reliable for real-world applications.
Support Vector Machines: Dividing Data with Best Gap
Support Vector Machines divide data using the best gap. They find the hyperplane that separates two classes most effectively. This maximizes the margin between the closest data points of different classes, leading to a clear division of data.
This approach optimizes data categorization, making Support Vector Machines an effective algorithm. They can handle multidimensional data and classify data points into different categories with high accuracy and efficiency.
Support Vector Machines are useful for complex data patterns and large datasets, as well as when there is a clear separation in the data. This makes them valuable across different domains, like medical diagnostics and stock market forecasting. They are capable of handling both linear and non-linear classification problems.
K Nearest Neighbors: Voting from the Nearest Points
The K Nearest Neighbors (KNN) algorithm uses “voting from the nearest points” to make predictions. It looks at the class of the majority of the K nearest data points to a given observation. The value of K determines the number of nearest neighbors used for voting. A smaller K gives more weight to the nearby data, while a larger K has a smoother effect and gives more weight to the overall data pattern.
The choice of K significantly affects the KNN algorithm’s accuracy and computational complexity. KNN is commonly used in pattern recognition, statistical estimation, and anomaly detection, especially in healthcare, finance, and social sciences. Its simple implementation and versatile applications make KNN a valuable tool for data classification and regression tasks.
Getting Smarter Together: Ensemble Learning Algorithms
Ensemble learning algorithms work by combining multiple models to improve prediction accuracy. They use the collective intelligence of several models to make more accurate predictions than any individual model.
Using ensemble learning algorithms minimizes the risk of overfitting and reduces prediction errors, resulting in more robust and accurate predictions compared to a single model approach in machine learning.
Common types of ensemble learning algorithms include:
- Bagging: Involves training multiple models independently and combining their predictions.
- Boosting: Focuses on training models sequentially to correct errors made by previous models.
- Stacking: Combines the predictions of multiple models using a meta-model to generate the final prediction.
These approaches offer a diverse set of methods to improve prediction accuracy in machine learning.
Combining for Strength: Gradient Boosting
Gradient boosting is a machine learning technique that builds strong predictive models. It combines multiple weak predictive models into a single strong model through an ensemble method. By iteratively training new models to minimize a loss function, gradient boosting enhances machine learning algorithms. This improves their ability to generalize and make accurate predictions on new data.
XGBoost, or Extreme Gradient Boosting, offers speed and performance optimizations, contributing to ensemble learning algorithms. It’s known for its efficiency and accuracy, making it a popular choice in various real-world machine learning problems. With the ability to handle missing data and prevent overfitting, XGBoost is important for overcoming the limitations of traditional gradient boosting. It greatly contributes to the advancement of ensemble learning techniques in AI algorithms.
XGBoost: Extreme Gradient Boosting
XGBoost, or Extreme Gradient Boosting, is a powerful distributed gradient boosting library. Its efficiency, flexibility, and portability set it apart. This library is widely recognized for its exceptional speed and performance, often used in machine learning competitions.
The way XGBoost works is by adding predictors sequentially to an ensemble, with each new model correcting its predecessor’s errors. This approach leads to continuous improvement in predictive accuracy.
Compared to other machine learning and deep learning algorithms, XGBoost stands out for its computational speed and model performance. It is particularly effective in handling large datasets and managing missing data. Furthermore, XGBoost’s versatility is evident in its ability to be combined with other models to create formidable ensemble methods.
In real-world applications, XGBoost’s scalability and overall accuracy make it a highly sought-after choice.
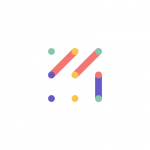
Vizologi is a revolutionary AI-generated business strategy tool that offers its users access to advanced features to create and refine start-up ideas quickly.
It generates limitless business ideas, gains insights on markets and competitors, and automates business plan creation.