Building Blocks of AI Startups’ Tech
Artificial intelligence (AI) startups are leading the way in technology innovation. They create solutions with the potential to revolutionize industries. Central to their success is their tech – the building blocks that drive their AI applications.
From machine learning algorithms to natural language processing tools, these technologies form the backbone of AI startups’ offerings. Understanding these tech components is essential for anyone interested in AI startups.
In this article, we will explore the fundamental elements that make up the tech of AI startups.
What Makes Up a Cool AI Tool?
An effective generative AI tool has important layers in its architecture. These include data processing, generative model, feedback and improvement, deployment and integration, application, data platform, orchestration, model, and infrastructure layers.
These layers work together to process data, generate models, provide feedback for improvement, and deploy and integrate the application with data platforms, orchestration, models, and infrastructure. The building blocks of AI tools, like data processing, generative models, and feedback and improvement, are crucial for ensuring the safety and effectiveness of AI’s smart choices.
Choosing the best AI tool for a project involves considering compatibility and security. This means evaluating the tool’s ability to process data effectively, generate accurate models, and provide seamless feedback and improvement in a secure manner to guarantee its compatibility and security within the project.
The Smart Layers of AI Tech
The ‘Brain’ Layer: Where the Thinking Happens
The ‘Brain’ layer is at the core of AI technology. It enables thinking and decision-making in generative AI models. This layer processes data, generates models, gives feedback for improvement, and manages the entire architecture.
By integrating components like data processing, generative models, deployment, and integration, the ‘Brain’ layer is crucial in developing and deploying AI models. It also includes large language models (LLMs), text-to-image models, and VAE, expanding generative models beyond text and images.
The ‘Talk and Listen’ Layer: Chatting With the AI
The ‘Talk and Listen’ layer in AI technology is important. It acts as a bridge between the AI system and the user. This layer allows users to interact with AI tools using natural language processing and speech recognition. This enables seamless communication and information exchange.
By using this layer, users can have better experiences. They can easily communicate their needs and preferences to AI systems, and receive tailored responses and solutions.
Improvements to the ‘Talk and Listen’ layer can make AI interactions more natural and effective. Advanced natural language understanding and generation capabilities can be included. For example, integrating sentiment analysis and context-aware responses can help AI systems understand user emotions and intentions. This leads to more personalized and empathetic interactions.
The ‘Getting Better’ Layer: How AI Learns
The ‘Getting Better’ layer in generative AI startups architecture helps AI learn and improve. It uses feedback loops and continuous improvement to enhance AI’s performance. Techniques like reinforcement learning, transfer learning, and unsupervised learning allow AI to learn from past actions. This leads to more accurate and effective outcomes. The ‘Getting Better’ layer significantly impacts the overall functionality and capabilities of AI tools.
It enables them to adapt to new data, optimize performance, and generate more realistic and diverse outputs. Moreover, this layer contributes to the scalability and reliability of AI models. It ensures they can efficiently handle complex tasks and deliver high-quality results.
The ‘Going Public’ Layer: Sharing AI With the World
Generative AI startups need to consider several important factors when sharing AI with the world through the ‘Going Public’ Layer.
They must ensure that the AI tools are safe, ethical, fair, private, and compliant with legal regulations. This involves implementing strong data privacy and security measures, as well as ethical guidelines for the development and deployment of AI models.
Organizations can ensure the safety and ethical use of AI tools by:
- conducting thorough testing and validation,
- obtaining necessary certifications, and
- involving diverse teams with different perspectives in the development process.
Steps can also be taken to ensure that AI tools are fair, private, and compliant with legal regulations when being shared with the world. This includes:
- implementing transparency and explainability features,
- obtaining consent for data usage, and
- adhering to industry-specific regulations, such as GDPR in Europe and HIPAA in the healthcare industry.
These measures are important for establishing trust and credibility when sharing AI tools with the public.
The Building Blocks of AI Tools
AI’s Many Talents Beyond Words and Pictures
Generative AI architecture goes beyond text and images. It includes layers for data processing, generative models, feedback and improvement, deployment and integration, application, data platform, orchestration, model, and infrastructure.
AI learns and improves its capabilities through large language models, text-to-image models, fine-tuning LLMs and text-to-image models, and VAE.
AI’s talents extend to video and music generation, showcasing its potential beyond traditional mediums.
With these advancements, AI exhibits its wide-reaching applications beyond just words and pictures, making an impact in various industries and creative fields.
Making Sure AI’s Smart Choices Are Safe
AI technology needs to be designed with safety and ethical considerations in mind. This ensures that its smart choices are secure for users and the public.
Measures to guarantee this include:
- Implementing strict ethical guidelines and standards in AI development.
- Emphasizing transparency, accountability, and fairness in algorithmic decision-making processes.
- Conducting rigorous testing, validation, and verification of AI models and algorithms.
- Prioritizing the development of explainable and interpretable AI systems.
- Continuous monitoring and auditing of AI systems to identify and address potential biases, errors, and vulnerabilities.
AI on the Job: Doing Work for Big Companies
AI is being used more by big companies for various tasks like customer service and data analysis. This can make work processes more efficient and productive. However, there are challenges to consider when using AI, such as the possibility of bias in decision-making and the importance of handling data properly for effective AI.
It’s also important for companies to ensure the safety, fairness, and privacy of AI tools in the workplace, particularly when handling sensitive customer data and making decisions that could affect employees. Companies can establish strict privacy protocols and form diverse AI development and oversight teams to create a fair and safe AI work environment. Considering these factors helps big companies use AI effectively while upholding ethical and legal standards.
Picking the Best AI Brain for Your Project
What Does Your Project Need?
Generative AI startups need a tool that meets specific project requirements. The AI tool must process data effectively, create generative models, provide feedback and continuous improvement, facilitate deployment and integration, and support various applications.
It should also operate across different layers including the data platform, orchestration, model, and infrastructure layers. The cost of implementing such an AI tool can vary based on the project’s specific needs, including factors like data processing, generative model sophistication, and integration complexity.
To ensure project security, the AI tool must have robust data privacy and security measures such as encryption, access controls, and secure data storage practices to prevent unauthorized access or data breaches.
How Much Will It Cost?
The cost of implementing an AI tool for a specific project can vary based on several factors. These factors include:
- The complexity of the project.
- The scale of the AI tool.
- The level of customization required.
For example, a simple AI tool for basic data processing may cost less than a more advanced AI tool for complex generative modeling and deployment. Additionally, different types of AI tools, such as natural language processing or image recognition, may come with different cost options based on their specific functionalities and capabilities.
Furthermore, the overall cost of using AI technology is influenced by factors such as data storage and processing requirements, hardware and infrastructure needs, as well as ongoing maintenance and improvement expenses.
Therefore, organizations considering the implementation of generative AI startups architecture should carefully evaluate these cost considerations to make informed decisions aligned with their budget and project requirements.
Keeping Your Secrets Safe
In generative AI startups, it’s crucial to keep your secrets safe. One way to do this is by using strong encryption techniques. This means making sure sensitive data is encrypted when it’s stored and when it’s being sent. By doing this, you can make sure that unauthorized people can’t get to your confidential information.
It’s also important to control who has access to the data. By only letting authorized people in and using multi-factor authentication, you can reduce the risk of data breaches.
But there are risks to consider too. One big risk is insider threats. This is when employees or trusted individuals accidentally or deliberately reveal sensitive information. To lower this risk, it’s important to have strong security policies and training programs for employees. This can help create a culture of data protection and security awareness.
On top of this, the world of cybersecurity is always changing, which means there’s always a risk to keeping your secrets safe. New types of attacks and smart hacking methods can put your data at risk. Companies need to stay updated on the latest security measures and technologies to guard against these external threats.
Tweaking AI to Make It Just Right
Generative AI startups work with complex architecture. This includes layers like data processing, generative models, feedback and improvement, deployment and integration, application, data platform, orchestration, and infrastructure.
For a cool AI tool, these key elements could be fine-tuning LLMs, text-to-image models, and VAE.
When tweaking AI for a specific project, startups can adjust parameters of generative models. This makes them more suitable for the desired outputs, like generating specific types of images or texts. This may involve adjusting training data, model architecture, or hyperparameters.
The AI needs to be easily integrated with existing technology for usability. This can be achieved by designing APIs or SDKs. These allow seamless integration with other systems, enabling generative AI models to be used alongside other tools and technologies.
Can the AI Get Along With Your Other Tech?
Generative AI architecture in 2022 faces a challenge when integrating with existing technology. The AI needs to work well with other tech components like data processing and infrastructure layers.
Potential challenges include compatibility issues, conflicting data formats, and varying processing speeds. To ensure smooth integration, steps can be taken such as thorough testing of the AI’s interaction with existing tech, standardized data formats, and flexible APIs for communication between components.
Clear communication between the AI development team and the existing tech team, along with regular updates and maintenance, are important to address compatibility issues and foster a good relationship between the AI and other technological components.
Oops! When AI Tools Might Cause Trouble
When the AI Makes You Look Bad
When the AI Makes You Look Bad, it’s important to avoid potential negative consequences.
Implement robust data processing and feedback mechanisms in the architecture.
This ensures that the generative AI model learns from diverse and inclusive datasets, reducing the risk of biased or unfair outcomes.
Strategies such as privacy-preserving data platforms and orchestration layers also play a crucial role in safeguarding user privacy and ensuring ethical AI practices.
Businesses can stay on the right side of the law by adhering to strict regulatory requirements and compliance standards when utilizing AI tools that have the potential to cause trouble.
By integrating transparent and interpretable AI models, businesses can mitigate legal risks and maintain trust with both customers and regulatory authorities.
Staying on the Right Side of the Law
When working with generative AI in startups, it’s crucial to consider the legal implications of utilizing AI tools to avoid potential legal issues. Businesses and individuals must ensure they comply with data protection regulations, intellectual property laws, and ethical guidelines when using AI technology.
For example, when generating content using AI, it’s important to respect copyright laws and ensure that the content produced does not infringe on existing intellectual property rights. Failing to stay on the right side of the law when using AI tools can lead to legal repercussions such as lawsuits, fines, and damage to the company’s reputation. Therefore, it’s essential for startups to have clear policies and procedures in place to ensure compliance with laws and regulations when utilizing generative AI technology.
This includes implementing robust data management practices, obtaining consent for data usage, and regularly monitoring and updating their AI systems to align with legal requirements.
Being Sure the AI Is Fair and Private
To make sure AI technology is fair and unbiased, Generative AI startups can take several steps:
- They can start by using diverse and inclusive datasets to train their AI models. This helps ensure that the data accurately represents all demographics.
- Additionally, they can use fairness metrics to evaluate their AI systems and identify any bias in their decision-making processes.
- To protect individuals’ privacy when using AI tools, companies can implement privacy-preserving techniques such as federated learning, differential privacy, and secure multi-party computation. These strategies allow AI models to learn from decentralized data sources without compromising users’ privacy.
- Generative AI startups can also monitor and regulate the fairness and privacy of their AI technology by establishing clear guidelines and standards for ethical AI development.
- They can collaborate with regulatory bodies and industry experts to ensure that their AI systems comply with privacy laws and uphold ethical standards.
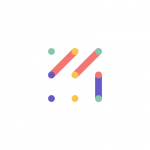
Vizologi is a revolutionary AI-generated business strategy tool that offers its users access to advanced features to create and refine start-up ideas quickly.
It generates limitless business ideas, gains insights on markets and competitors, and automates business plan creation.