Simple Guide to Text Generation Analysis
Text generation analysis is a powerful tool. It reveals valuable insights about written content. By examining patterns and trends in text, we can gain a deeper understanding of language and communication.
In this simple guide, we will explore the basics of text generation analysis. We’ll also learn how it can be used to unlock the hidden meanings within written work.
Whether you’re a student, writer, or researcher, this article will provide you with the knowledge and skills to harness the power of text generation analysis.
What Is Text Creation with AI?
Text creation with AI involves using artificial intelligence models to automatically generate human-like text. These models are designed to employ rule-based techniques, language models, and deep learning approaches to produce natural language text.
The importance of text creation with AI lies in its wide range of applications, including natural language processing, machine translation, summarization, and dialogue systems. People should be aware of this technology as it has the potential to revolutionize various industries and improve efficiency in tasks that involve large amounts of text.
AI is trained to create text through pre-training and fine-tuning processes. These processes are necessary to address challenges such as biases in generated texts and the need for semantic consistency and high-quality training data.
Making Sense of Text Creation: The Basics
Understanding the basics of text creation with AI is important for people who work with natural language processing. This includes machine translation, summarization, and dialogue systems. AI helps create high-quality training data and address biases in generated texts. It also ensures semantic consistency and supports pre-training and fine-tuning processes, which are important for the success of text generation models.
Preparing data for AI to learn in text creation involves using rule-based techniques, language models, and deep learning approaches. This helps AI understand the structure and context of human language. Large datasets expose AI to diverse and extensive language patterns, aiding in generating human-like text.
AI generates coherent sentences for text creation through methods like language modeling, which involves predicting the next word in a sequence of words. By analyzing and learning from large amounts of text data, AI can automatically generate contextually relevant and linguistically coherent sentences. This ability is fundamental to developing sophisticated language models like GPT-3, BERT, ALBERT, and T5, showcasing the power of AI in text generation analysis.
Text Creation: Why We Should Care?
AI is important for text creation. It enables the automatic generation of human-like text, which has numerous applications in natural language processing, machine translation, summarization, and dialogue systems.
AI text creation models can significantly improve language generation and understanding processes. The potential benefits and implications of AI text creation are vast. It can enhance the efficiency and accuracy of language-related tasks, leading to improved communication, information dissemination, and even creative writing.
AI text creation can impact language, communication, and information dissemination. It raises important considerations about biases in generated texts, semantic consistency, and high-quality training data.
Addressing these effects is crucial to ensuring that text creation with AI does not perpetuate harmful biases or misinformation. Understanding and addressing these effects can lead to more effective and ethical use of AI in language-related processes. Therefore, paying attention to the potential impacts of AI text creation on language, communication, and information dissemination is essential.
Getting Set Up to Create Text
Finding AI Tools: Where to Start
When starting the search for AI tools for text creation, it’s important to consider specific application needs, the technical expertise required, and the scalability of the tools. Different resources and platforms are available to find AI tools. These include open-source libraries, online marketplaces, and specialized AI software vendors.
To ensure that the AI tools found are reliable and effective for text creation purposes, users can look for features such as pre-trained models, flexible APIs, and extensive documentation and community support. Reviewing case studies and user testimonials can provide valuable insights into AI tools’ real-world performance and usability.
By carefully evaluating these factors and utilizing a combination of resources, individuals and organizations can take the first steps in finding AI tools for text creation that best align with their needs and goals.
Preparing the Data for AI to Learn
Data preparation is a crucial step in ensuring that AI can effectively learn. To best prepare the data for AI, it should be structured and organized in a way that is easily understandable and accessible.
This includes labeling data, breaking it down into smaller segments, and ensuring that it is in a format that AI models can easily process, such as text or numerical data. Steps should also be taken to clean and preprocess the data, such as removing irrelevant or duplicate entries, handling missing data, and normalizing or standardizing the data for consistency and accuracy.
Addressing potential biases or issues in the data is also essential before AI learning begins. This involves identifying and mitigating any biases present in the data, which could lead to skewed results or inaccurate predictions.
It also includes ensuring that the data is representative and inclusive, without any discriminatory or misleading information that could negatively impact the AI’s learning process. By structuring, cleaning, and addressing biases in the data before AI learning begins, the overall quality and accuracy of AI-generated text can be greatly improved.
Training the AI: Teaching it About Words
Learning Phase: AI Starts to Understand
During the learning phase, AI can understand and learn from text. It uses methods like rule-based techniques, language models, and deep learning approaches. These methods help AI process and understand the data. They do this by analyzing patterns, identifying word relationships, and predicting the next words in a sequence.
AI can also be improved and fine-tuned during the learning phase. This involves pre-training and fine-tuning processes, exposing the model to diverse text data. Then, its parameters are refined to improve its understanding and generate human-like text. These processes help address challenges like biases in texts, semantic consistency, and the need for high-quality training data.
Improving AI with Practice: Fine-Tuning
Fine-tuning is important for improving AI performance in text creation. It involves adapting a pre-trained language model to specific datasets, allowing it to generate more precise and relevant text. This practice helps minimize biases, improve consistency, and create high-quality content tailored to specific tasks and domains.
Making Text: How AI Turns Learning into Sentences
Helping the AI Get Better and Better
Researchers can use pre-training and fine-tuning processes to improve the AI’s understanding of language and its ability to generate coherent text. These processes involve exposing the AI to diverse language data to learn various linguistic patterns. Methods such as rule-based techniques, language models, and deep learning approaches can enhance the AI’s text creation capabilities.
This improves the AI’s language processing and generation, enabling it to produce more natural and appropriate text. To avoid biases in text generation, researchers can focus on developing evaluation metrics and high-quality training data, minimizing potential biases in the AI’s generated content.
Solving Problems: When AI’s Words Get Mixed Up
Bias in Words: How AI Can Mistake
Bias, in other words, can significantly impact AI’s interpretation and generation of text. For instance, if the training data for a language model contains biased or prejudiced language, the AI system could inadvertently generate biased content. This can lead to potential consequences such as the perpetuation of stereotypes, discrimination, or misinformation in the texts created by AI.
To mitigate this, AI can be trained to recognize and avoid bias in words during text creation by using high-quality, diverse training datasets and incorporating techniques for bias detection and debiasing. By recognizing and addressing bias in the training data, AI systems can learn to produce more accurate, fair, and inclusive text outputs.
AI’s Big Appetite for Lots of Words to Learn From
AI’s big appetite for many words to learn from is significant in text creation. The more words AI has access to, the better it can understand and interpret language, leading to more coherent and meaningful text generation. By continuously learning from many words, AI can improve its language processing capabilities, leading to more accurate translations, better summarizations, and enhanced dialogue systems.
This extensive learning also allows AI to recognize semantic nuances better, improving the overall quality of the generated text. Moreover, AI’s continuous learning from a vast collection of words can be leveraged to improve text creation by ensuring that the generated content is free from biases and maintains semantic consistency.
The Future of Text Creation with AI
New Paths for AI Learning Languages
AI is making language learning and text generation easier. With models like GPT-3, BERT, ALBERT, and T5, AI can create human-like text in different languages. It uses rule-based techniques, language models, and deep learning approaches. AI technology has also improved pre-training and fine-tuning processes to enhance language learning and text creation.
Recent AI research addresses challenges such as biases in generated texts, semantic consistency, and high-quality training data. The goal is to ensure that AI-generated text is accurate, culturally sensitive, and coherent across languages and contexts. The future of AI in language learning and text generation looks promising. Ongoing research emphasizes advancements in language models for natural language processing, machine translation, summarization, and dialogue systems.
Being Fair: Keeping AI’s Text Nice for Everyone
Creating fair and inclusive AI text involves considering diverse perspectives and backgrounds. This means training text generation models on various data sources to capture different viewpoints, experiences, and cultural nuances. Large-scale datasets representing various demographic groups can help ensure respectful and considerate AI-generated text. Detecting and addressing bias during training and fine-tuning processes is essential to mitigate potential stereotypes or prejudices.
Collaborating with domain experts, ethicists, and community representatives to develop guidelines and best practices can promote fairness and respect in AI-generated content. These measures enhance the text generation process to maintain fairness and inclusivity for all users.
AI and Pictures: Using More Than Just Words
AI has changed how we use images in text. It goes beyond words by using image recognition, improving the text generation process. This creates the potential for AI to use pictures in text creation, making detailed narratives based on visuals. AI and pictures can also help language learning. AI models can analyze images and generate descriptive text to aid understanding, enhancing text quality and offering a better learning experience.
Combining AI and pictures in text opens up exciting possibilities for how we interact with visual and textual information.
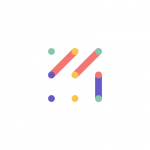
Vizologi is a revolutionary AI-generated business strategy tool that offers its users access to advanced features to create and refine start-up ideas quickly.
It generates limitless business ideas, gains insights on markets and competitors, and automates business plan creation.