Tech Talk: Variable Pricing Algorithms
Variable pricing algorithms are becoming more common in technology and commerce. These algorithms analyze real-time data to adjust prices based on factors like demand, competition, and customer behavior.
In this article, we’ll explore how these algorithms work, their impact on consumers and businesses, and the ethical considerations surrounding their use. If you’ve ever wondered why prices seem to change unexpectedly, keep reading to learn about variable pricing algorithms.
History and Evolution of Dynamic Pricing Strategies
Dynamic pricing strategies have changed a lot over time. They adapt to market changes and use new technology. Pricing algorithms help companies adjust prices in real-time based on different factors. This helps them make the most profit and meet market demand.
Pricing algorithms are a big deal. They let businesses use AI and machine learning to predict market trends and set prices better.
Different ways of doing dynamic pricing have helped it grow. Things like Bayesian models, reinforcement learning, and decision trees have made it possible. These models let businesses look at things like supply and demand, what their competitors are charging, and how customers behave. This helps them find the best price. They can also do personalized pricing, time-based pricing, and auction-based pricing.
But, using dynamic pricing can cause some problems. Customers might feel left out, lose trust in the company, and think the brand isn’t great. Changing prices a lot makes customers wonder about the company’s intentions and the value of its stuff. So, businesses need to tell people why they’re using pricing algorithms, set rules for them, and keep an eye on how they affect customers. This way, they can step in if needed and keep customers happy.
Understanding How Variable Pricing Algorithms Operate
Basic Functioning of Dynamic Pricing Systems
Variable pricing algorithms in dynamic pricing systems use AI and machine learning to analyze different factors. These include market conditions, customer behavior, supply and demand, and competitor pricing. The goal is to find the best price. These algorithms use real-time data to adjust prices immediately based on market trends and demand.
The main factors that affect price changes in dynamic pricing systems are supply and demand, delivery time, competitor prices, and customer behavior. By analyzing these factors constantly, the algorithm can find the best price for products or services. This helps the business meet its goals and increase revenue while keeping customers happy.
Dynamic pricing systems have basic functions including time-based pricing, segmented pricing, personalized pricing, and auction-based pricing. These functions let businesses adjust pricing in real-time based on different factors and customer segments. The main aim is to make profits and optimize pricing.
Key Factors Influencing Price Adjustments
Price adjustments in dynamic pricing systems are influenced by supply and demand, competitor prices, and external events. Algorithms are used to analyze these factors and set the best price in real-time.
Consumer behavior trends are important in the decision-making process for price adjustments. Dynamic pricing systems consider customer behavior patterns, preferences, and purchasing history to optimize pricing.
To reduce risks with variable pricing, companies can implement strategies like developing proper use cases for algorithms, assigning an owner to manage them, setting pricing guardrails, and stepping in to override automation when needed. These measures give companies control over how dynamic pricing affects customer relations and brand reputation.
Various Approaches to Dynamic Pricing
Bayesian Pricing Models
Bayesian pricing models use historical data to determine the optimal price. This statistical model updates beliefs based on new evidence through Bayesian inference, leading to better pricing decisions. When adjusting prices, these models consider demand elasticity, competitor pricing, market trends, and customer segmentation.
By using AI and machine learning, they can analyze complex data to identify consumer behavior trends and adjust prices in real-time based on patterns like purchase frequency, time of purchase, and price sensitivity. Businesses can align their pricing strategies with customer preferences and market dynamics while maximizing revenue and staying competitive by leveraging Bayesian pricing models effectively.
Applying Reinforcement Learning for Price Optimization
Reinforcement learning can be effectively applied to optimize pricing in dynamic pricing systems by enabling algorithms to learn and adapt to market conditions in real-time. The use of reinforcement learning allows pricing algorithms to make pricing decisions based on past experiences and feedback, thereby maximizing profits while minimizing the risk of alienating customers.
Key factors influencing price adjustments when using reinforcement learning for price optimization include customer behavior, competitor pricing, and market demand. These factors provide valuable insights that influence pricing decisions, ensuring that the algorithm adapts to changing market conditions. Moreover, decision trees can be effectively utilized in pricing strategies by providing a structured framework for the algorithm to analyze different pricing scenarios.
This allows businesses to make informed decisions based on the various factors that influence pricing, ultimately leading to better price optimization and improved customer relations.
Utilization of Decision Trees in Pricing
Decision trees are used in pricing to optimize price adjustments. They analyze key factors like customer behavior, competitor pricing, and demand patterns. This helps businesses determine the best price for their products in real time, aligning with market trends and maximizing revenue.
Additionally, decision trees can create personalized pricing structures. This considers individual customer preferences, purchase history, and timing, leading to tailored prices and increased customer satisfaction.
Advantages of using decision trees in pricing strategies include improved price optimization, enhanced customer experience, and adaptability to changing market conditions. Challenges may arise in capturing dynamic market trends and maintaining pricing relevance in a fast-paced business environment.
Time-Sensitive Pricing Structures
Time-sensitive pricing structures can adapt prices to consumer behavior trends. This is done by utilizing dynamic pricing algorithms. These algorithms analyze and forecast market trends in real-time.
As a result, businesses can adjust prices based on factors such as supply and demand, competitor prices, and customer behavior. Additionally, these algorithms can optimize price adjustments based on external events. This enables businesses to react swiftly to changes in market conditions.
Segmentation-based pricing tactics can be implemented effectively by tailoring prices to specific customer segments. This ensures that each group receives offers and discounts personalized to their needs and preferences.
By leveraging dynamic pricing models, businesses can effectively implement time-sensitive pricing structures. They do this to stay competitive, drive revenue, and enhance customer satisfaction.
Consumer-Centric Personalized Pricing
Consumer-centric personalized pricing strategies can be effectively implemented by using variable pricing algorithms. These algorithms prioritize individual customer needs and preferences. They analyze customer behavior, purchasing history, and demographics to tailor pricing and promotional offers to specific individuals.
Businesses need to consider key factors such as supply and demand, competitor pricing, customer loyalty, and the perceived value of products or services to determine price adjustments based on consumer behavior and segmentation.
To mitigate potential risks and challenges associated with personalized pricing, businesses should establish proper use cases and narratives for implementing algorithms. They should also assign dedicated management, set and monitor pricing guardrails, and intervene promptly to override automation when necessary. This is important in order to preserve customer relations and minimize any negative impacts.
Segmentation-Based Pricing Tactics
Segmentation-based pricing tactics involve tailoring pricing strategies to specific customer segments. This is done by categorizing customers based on their purchasing habits, demographics, or preferences.
For example, an e-commerce platform may offer student discounts, seasonal promotions for young parents, or premium memberships for frequent shoppers.
Implementing these tactics can lead to benefits such as increased customer loyalty, improved satisfaction, and capturing a larger share of customer spending. However, challenges include ensuring fairness and transparency, avoiding customer alienation, and managing price discrimination concerns.
It’s important to strike a balance between maximizing profitability and maintaining positive customer relationships when using segmentation-based pricing tactics.
Advantages of Implementing Dynamic Pricing Models
Enhanced Profit Maximization
Dynamic pricing systems can help businesses maximize profits by adjusting prices in real time based on market conditions and demand. They use algorithms to analyze factors like supply and demand, competitor prices, customer behavior, and external events to determine the best price for maximizing revenue and minimizing customer churn.
Implementing dynamic pricing models has several advantages. It allows businesses to compete with competitors’ prices, improve customer experience, and align with business objectives. Dynamic pricing models also enable businesses to use various pricing strategies, such as time-based pricing, segmented pricing, personalized pricing, and auction-based pricing, to optimize profits further.
To maximize profit, businesses should develop a use case and narrative for implementing algorithms, assign an owner to manage them, set and monitor pricing guardrails, and act quickly to override the automation when necessary. These practices ensure that dynamic pricing strategies effectively contribute to profit maximization without undermining customer loyalty and brand reputation.
Adapting Prices to Consumer Behavior Trends
Companies can change their prices to match how people are buying things right now. They can do this by using special computer programs that learn and make decisions on their own, based on data. These programs look at things like how much of a product is available, what other companies are charging, how people are acting, and things happening in the world. This helps the companies set the best price at any given time.
And when they adjust their prices, they should think about how it might make customers feel. This includes how it might affect their emotions and their loyalty to the company. They should also think about how customers see the company and how they believe in the value of the products. But, there are risks. Changing prices too much could make customers upset, make them less loyal, and hurt the way people think of the company.
To deal with these risks, companies should have a good plan and story for using these special programs, put someone in charge of them, set and watch over rules for prices, and be ready to step in if needed.
Best Practices in Variable Pricing
Setting Clear Business Objectives for Pricing Strategies
Setting clear business objectives for pricing strategies is important. This includes maximizing revenue, reducing customer churn, competing with rivals, improving customer experience, and aligning pricing strategies with overall business goals.
To evaluate the success of pricing strategies, companies can use metrics such as revenue generated, customer satisfaction, and market share. When choosing pricing methods, companies should consider their business objectives, customer segmentation, market trends, product value, and competition.
These considerations are important for aligning pricing strategies with larger company goals and maximizing the effectiveness of variable pricing algorithms.
Determining Metrics for Success Evaluation
Successfully evaluating the implementation of dynamic pricing strategies involves considering various factors. Market trends, customer behavior, and competitor pricing are important in achieving pricing optimization. These metrics help companies gauge the appropriateness of their pricing, reflecting demand and staying competitive.
Aligning pricing metrics with overall business objectives is crucial. For example, prioritizing metrics that minimize customer churn and enhance customer experience is key. Accurate data accumulation and analysis is fundamental in evaluating pricing strategies.
Using AI and machine learning algorithms allows businesses to gather and analyze large sets of data, including variables like supply and demand, customer behavior, and competitive pricing. This comprehensive approach helps companies accurately measure the success of their dynamic pricing strategies.
Choosing an Appropriate Pricing Method
When choosing a pricing method, it’s important to consider demand forecasts, competitor prices, and customer behavior.
These factors help in setting optimal prices, maximizing revenue, and avoiding customer churn.
To align the chosen pricing method with business goals, implementing advanced analytics and AI tools for real-time market analysis and customer preferences is effective.
To mitigate risks associated with variable pricing, setting clear guidelines and boundaries for the pricing algorithm is helpful in preventing extreme price fluctuations.
Having a dedicated owner to monitor and manage pricing changes, and intervene when necessary, can help avoid negative effects on customer relations due to constant price changes.
Accumulating Relevant Data for Pricing Analysis
Businesses can gather relevant data for pricing analysis using several methods. These include employing web scraping tools to gather data from competitor websites, using customer relationship management systems to collect customer purchase history and preferences, and implementing surveys and interviews to gather direct feedback from customers.
To ensure the accuracy and comprehensiveness of the data for pricing analysis, businesses can implement data validation and cleaning processes. They can also use data from reliable and diverse sources and employ data analytics and machine learning tools to identify and eliminate inaccurate or outdated data.
Moreover, historical pricing data can be used to inform pricing analysis decisions by identifying pricing trends, understanding customer purchase behavior, and evaluating the impact of previous pricing changes on sales and customer satisfaction. It can also be leveraged to forecast demand and optimize pricing strategies for different seasons or customer segments.
Deciding Specific Price Points
Businesses can set specific price points for their products and services using dynamic pricing algorithms. These algorithms analyze real-time data and market trends to adjust prices. Factors like supply and demand, competitor prices, customer behavior, and external events are considered. Data and analytics support decision-making for pricing, as dynamic pricing algorithms use AI and machine learning to predict trends and optimize pricing.
They leverage models such as Bayesian, reinforcement learning, and decision tree models to analyze data and optimize pricing strategies. By using dynamic pricing, businesses can adjust prices based on market conditions and demand to increase revenue and enhance customer experience.
Strategic Development for Variable Pricing Algorithms
Aligning Pricing with Business Goals
Pricing strategies can be aligned with specific business goals and objectives. First, understand the desired outcomes, like revenue maximization, customer retention, or market share growth. Then, tailor variable pricing algorithms to optimize pricing in real-time to achieve these goals.
However, aligning pricing with business objectives has potential risks and challenges. These include customer alienation, loyalty erosion, and brand reputation damage caused by frequent price changes.
Variable pricing algorithms can help achieve business goals by using AI and machine learning to analyze factors like supply and demand, competitor pricing, customer behavior, and market trends. This data-driven approach ensures that pricing decisions align with the business’s specific objectives, maximizing revenue and improving overall customer experience without compromising customer loyalty.
Reducing Potential Customer Churn Through Fair Pricing
Fair pricing strategies help keep customers from leaving because of constant price changes. When customers feel that pricing is fair and stays the same, they are more likely to stay loyal. But, using fair pricing also comes with its own challenges.
For example, pricing algorithms may not consider how customers feel about frequent price changes. Also, dynamic pricing can make customers feel like the products are not worth as much. To make sure dynamic pricing helps the business and keeps customers, companies can create a plan for pricing algorithms. They can also have a manager oversee pricing algorithms, set limits, and step in when needed. This way, companies can control how dynamic pricing affects customers and keep good relationships with them.
Matching or Undercutting Competitor Prices
Businesses can set prices strategically to compete with or offer lower prices than their rivals without hurting profits. They can do this by using variable pricing algorithms. These algorithms help companies analyze different factors like demand, supply, and customer behavior to decide the best price in real-time. This way, businesses can make smart choices about when and how to adjust their prices to match rivals while still making the most money.
To keep up with competitors’ price changes, companies can use machine learning models like Bayesian, reinforcement learning, and decision tree algorithms. These models can find market trends and predict what rivals might do with their prices. This helps businesses adjust their prices quickly and stay profitable.
But there are risks in matching or beating competitor prices. This can make customers less loyal and hurt a company’s reputation if prices change too often. To avoid these risks, firms should stick to good practices for using dynamic pricing, assign someone to manage prices, set and watch over pricing limits, and step in manually if needed to keep customer relations on track. By following these strategies, companies can compete with or beat rival prices while keeping customer trust and staying profitable in the long run.
Challenges and Risks Associated with Variable Pricing
Mitigating the Impact on Customer Loyalty
To maintain customer loyalty when using variable pricing strategies, businesses can follow these steps:
- Establish a clear use case and story for algorithm implementation.
- Appoint an owner to oversee and manage the pricing algorithms.
- Set up pricing guardrails to monitor acceptable pricing ranges.
- Be ready to intervene when needed to override automation and consider customer needs.
- Communicate effectively about the reasons behind price changes, emphasizing value additions and service improvements.
- Prioritize transparent communication to maintain customer trust and minimize dissatisfaction.
These proactive strategies can help retain customers while adjusting pricing to stay competitive.
Managing Risks of Autonomous Pricing Decisions
Businesses can lessen the impact on customer loyalty by:
- Developing a clear use case and narrative for the implementation of variable pricing algorithms.
- Assigning a dedicated owner to manage the algorithms.
- Setting and monitoring pricing guardrails.
- Being prepared to override the automation when needed.
To handle the risks linked with autonomous pricing decisions, businesses can adopt dynamic pricing strategies like:
- Time-based pricing.
- Segmented pricing.
- Personalized pricing.
- Auction-based pricing
Implementing these strategies enables businesses to adjust prices in real-time based on market conditions and demand, while minimizing negative impact on customer relations.
It’s also recommended to align pricing with business goals and reduce potential customer churn by employing dynamic pricing algorithms. These algorithms leverage AI and machine learning to predict market trends, thus achieving pricing optimization. This approach ensures that pricing decisions align with business objectives and are in the best interest of customers.
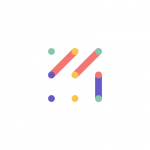
Vizologi is a revolutionary AI-generated business strategy tool that offers its users access to advanced features to create and refine start-up ideas quickly.
It generates limitless business ideas, gains insights on markets and competitors, and automates business plan creation.