Text Generation Architecture Explained
Text generation architecture is the foundation of many language-related AI tools we use daily, such as chatbots and auto-complete features. Understanding how this architecture works can help us understand the power and potential of language AI and its limitations and challenges.
This article will explore the inner workings of text generation architecture, breaking down the process into simple, easy-to-understand terms. By the end, you’ll have a clearer picture of how these language AI systems operate and their impact on our daily lives.
Getting Ready to Make a Machine Write
What We Need to Get Started
You’ll need some crucial tools to begin building a text generation model using the transformer architecture. These include TensorFlow, PyTorch, and Hugging Face’s Transformers library. These tools are essential for implementing the transformer architecture and providing the necessary frameworks, libraries, and pre-trained models.
Through web scraping, you can obtain extensive datasets from various online repositories to find stories from which the machine can learn. These repositories include Project Gutenberg, OpenAI GPT-4o, and platforms like Reddit or Wikipedia.
Once you have the stories, the following steps involve preprocessing the text data. This includes:
- Tokenization: Segmenting the text into words or subwords
- Dataset preparation: Splitting the text into training and validation sets
- Input embedding: Encoding the text into numerical vectors
- Positional encoding: Providing the model with information on the order of the words in the input
These steps are crucial for effectively preparing the stories for the machine to learn from. They ensure a thorough understanding of the text generation process using the transformer architecture.
Get the Right Tools: TensorFlow and More
Understanding the framework and its components—including tokenization, dataset preparation, input embedding, and positional encoding—is essential for implementing TensorFlow for text generation. Knowledge of Python and experience with deep learning models are also crucial.
Using TensorFlow as a tool can enhance machine learning capabilities for building and training text generation models. It can be combined with pre-trained language models, custom datasets, and open-source libraries to improve performance. These resources provide valuable pre-built components and datasets, expediting model development and boosting effectiveness.
Finding Stories for the Machine to Learn From
Training a text generation model involves gathering stories from different sources, such as books, articles, and written materials. These diverse stories should help the machine understand language and writing styles. It is helpful to include stories with dialogue, descriptive language, and various sentence structures. Once the stories are collected, they must be organized into a dataset for the machine to learn from.
This includes tokenization, input embedding, and positional encoding to help the machine effectively process and understand the stories. Focusing on a wide range of high-quality stories and preparing them organized can optimize the machine’s learning process to produce coherent and contextually accurate text.
Read and Get the Stories Ready
Finding and selecting stories for the machine to learn involves getting various texts from different genres and styles. These stories should be diverse to expose the machine to other forms of language and content.
Once the stories are selected, the next step is to prepare them for the machine to process. This includes cleaning the text to remove any irrelevant information and formatting the stories consistently and in an easily digestible manner.
This process is significant because it provides the machine with a rich and well-organized dataset to learn. The machine can better understand and generate coherent and relevant text by carefully curating and preparing the stories.
This step is crucial for enabling the machine to produce high-quality output using the transformer architecture for text generation.
Teaching the Machine the ABCs of Stories
Turning Words into Numbers
Text generation architecture involves turning words into numbers. This is a critical step in training machines to understand and generate text.
Tokenizing plays a crucial role. It helps break down text into smaller components, such as words, subwords, or characters, allowing the machine to understand the text and its context.
To train the machine effectively, groups of training stories are created. These provide a diverse range of examples for the machine to learn from. This ensures that the machine can recognize different patterns and styles in the text, leading to more accurate and varied text generation.
For instance, text generation models using the transformer architecture use tokenization to convert words into vector representations. This makes it easier for the machine to process and generate coherent text.
By providing diverse training stories, the machine can learn to generate contextually and grammatically accurate text, enhancing its overall text generation capabilities.
Understanding the Text: Tokenizing
Tokenizing is breaking down a piece of text into smaller units, typically words or subwords, known as tokens. It’s essential for understanding the text, and it allows a machine to interpret and analyze the words in a story.
In text generation, tokenizing is used to turn words into numbers. This process, called vectorization, helps a machine learn from them. By breaking down the text into tokens, each word can be represented by a specific number. This enables a machine learning model to process the information and generate new text based on the learned patterns.
Additionally, tokenizing helps in creating training datasets for machines. It does this by grouping specific stories or texts based on their tokens. This allows the machine to learn from a variety of inputs. This grouping of tokens is essential for teaching a machine to generate coherent and contextually accurate text based on the input it has received.
Making Training Examples and Targets
Several steps are involved in creating training examples and targets for a machine-learning model.
First, collect and organize the dataset of stories or texts.
Then, tokenize and encode the stories to create input sequences for the model.
Shift the input sequences by one token to create target sequences. These targets are the ground truth for the model’s prediction of the next token.
When creating groups of training stories, it’s important to consider diversity in topics and writing styles. This helps the model learn a wide range of linguistic patterns.
Also, the length of the stories should be considered to prevent bias in the model.
By creating a balanced dataset, the machine learning model is exposed to different types of content and can generate more accurate and diverse text.
For example, when training a text generation model, use stories from different genres, such as news articles, fiction, and scientific papers. This ensures the model captures various writing styles and vocabulary.
Making Groups of Training Stories
When creating training sets for text generation, it’s essential to include various stories, topics, and writing styles. This helps the machine learn to produce diverse and realistic text.
For instance, training data can include news articles, short stories, and technical writing to expose the model to linguistic and structural patterns. Diverse training stories can be grouped using clustering algorithms or manual categorization based on content and style.
This approach ensures that the machine learns from a broad range of stories, enabling it to generate text across various genres and topics. To further enhance diversity, strategies like oversampling underrepresented categories and data augmentation techniques can be utilized to improve the model’s performance.
Building the Storyteller Machine
Making a Decoder Layer
You need multiple components to make a decoder layer. These components are self-attention, layer normalization, and feedforward neural networks.
The self-attention mechanism lets the decoder weigh different words in the input sentence. This helps it focus on the relevant words for generating the next word.
Layer normalization ensures that the input to each sub-layer has a mean of 0 and a standard deviation of 1. This helps stabilize the deep neural network’s training.
Also, feedforward neural networks help map the input space to an output space.
Different attention heads in the decoder layer can convey various ideas by focusing on other parts of the input sequence. This helps the model understand complex relationships within the input sentence, improving its text generation abilities.
Masking plays an essential role in enhancing the machine’s storytelling abilities. It prevents the decoder from peeking ahead during the text generation process. This encourages the model to generate each word based only on previous words, adding an element of suspense and coherence to the generated text.
Different Attention Heads for Different Ideas
Different attention heads in the text generation architecture help the machine focus on various aspects of the input text.
For instance, one attention head might focus on character relationships, while another might attend to setting and background details.
This diversity enables the machine to understand and generate diverse story ideas by capturing different narrative elements.
Leveraging multiple attention heads enhances the machine’s storytelling capabilities, allowing it to process a broader range of information and produce more nuanced and contextually rich stories.
Moreover, different attention heads can improve the machine’s ability to understand and generate diverse story ideas by capturing a broader array of concepts and themes, resulting in more engaging and imaginative narratives.
Therefore, using different attention heads in the text generation architecture enables the machine to produce a wider variety of compelling and well-rounded stories.
How the Machine Throws in Excitement: Masking
Masking is essential in text generation architecture. It helps the model focus on specific words or tokens during training, allowing the machine to learn the structure of the input data and generate coherent output.
The machine uses masking differently, like self-attention mechanisms and positional encodings. This helps it pay attention to different parts of the input text at various stages of learning. As a result, the machine can create well-structured and meaningful output, enhancing its storytelling abilities.
Getting the Machine Ready to Tell Tales
Setting Up the Story-writing Model
Setting up a story-writing model requires tools such as TensorFlow and PyTorch. These tools are essential for implementing the transformer architecture. They play a significant role in creating and training the text generation model.
In addition, tokenization, dataset preparation, input embedding, and positional encoding are essential to setting up the story-writing model. To find stories, one could use large datasets like books, articles, or other written materials. These materials can then be processed and prepared for the machine to learn from.
The machine must be trained to use these stories to improve its writing capabilities. This involves feeding the machine a large amount of text data and adjusting its parameters to ensure it learns to generate high-quality text.
The machine can be trained to write better and produce coherent, contextually relevant stories by taking these steps.
First Try: Will the Machine Write?
The “First Try: Will the Machine Write?” section discusses training a machine learning model for text generation, especially for storytelling. It covers transformer architecture, tokenization, dataset preparation, input embedding, and positional encoding. This helps prepare the machine to write stories by explaining the technical aspects of text generation. The code snippets use PyTorch to show how to use the transformer architecture.
This section is a beginner’s guide for developing generation models and demonstrates the practical use of the transformer architecture for storytelling.
Making the Machine Learn Better with Training
Training examples and targets can help the machine learn better. A diverse and balanced dataset representing different text aspects is essential. Techniques like data augmentation and oversampling can create a robust training set.
Clear and specific targets are needed for the model to enhance its learning process. This leads to more accurate and coherent text generation.
Optimizers and loss functions are essential tools. They enhance the machine’s learning during training. Optimizers like Adam and SGD adjust the model’s weights to minimize the loss function, which measures the difference between predicted and actual text. Fine-tuning the learning rate and using suitable loss functions like cross-entropy can significantly improve the model’s performance.
Customized training can make the machine a super-intelligent storyteller. Transfer learning methods and fine-tuning pre-trained language models on specific storytelling tasks can be leveraged. Exposing the model to diverse text data and using reinforcement learning techniques based on user feedback can help the machine generate engaging and contextually relevant stories.
Writing Tools the Machine Uses: Optimizer and Loss Function
The optimizer and loss function are like writing tools. The optimizer adjusts the model’s parameters to minimize the loss, which measures the difference between the model’s predictions and the actual output. Optimization algorithms like Stochastic Gradient Descent (SGD) or Adam help the machine improve its performance by iteratively updating the model’s weights.
The optimizer and loss function work together in the writing process to guide the machine in generating coherent and relevant text. They help the model understand language patterns and nuances, enabling it to produce natural and accurate writing. The optimizer and loss function are essential for the machine to produce effective writing, ensuring that the generated text is coherent, grammatically correct, and contextually appropriate. Without effective optimization and loss evaluation, the machine’s writing output may lack meaningful structure and relevance.
Therefore, the optimizer and loss function enable the machine to produce high-quality, contextually relevant text.
Let the Story Writing Begin!
Making the Machine Write Its First Story
Several steps are necessary to prepare a machine to write its first story.
- First, it must understand the transformer architecture, applications, and model components like tokenization and input embedding.
- The machine must also comprehend and interpret the text it will learn from, achieved through techniques like dataset preparation and positional encoding.
- Equipping the machine with the ability to implement code using platforms like PyTorch is also important.
- Training tools and techniques, such as natural language processing algorithms and language generation models, can help the machine become a proficient storyteller.
- Leveraging these technologies, the machine can generate coherent and engaging stories, marking an important milestone in text generation.
Saving Stories the Machine Writes
Text generation architecture allows for the efficient saving of machine-produced stories. This can be done using database, cloud, or text file formats.
Stories can be organized and stored for future use through chronological ordering, categorization by topic or genre, or sentiment analysis to group them according to emotional content.
These machine-generated stories can then be retrieved and used whenever needed, providing a valuable resource for various applications such as content generation, creative writing, or personal inspiration.
The flexibility of text generation architecture ensures that these stories are not only saved but also easily accessible and organized for efficient use, making them a valuable asset in artificial intelligence and content generation.
Making a Super Smart Storyteller
Teaching the Machine Tricks: Customized Training
Customized training helps machines learn specific storytelling techniques. This involves tailoring the training data to focus on those skills.
For example, curating a dataset with diverse storytelling styles exposes the machine to different narrative structures, character development methods, and plot devices. This targeted approach helps the machine identify patterns and develop the ability to generate text-matching desired storytelling techniques.
One effective strategy is using transfer learning. This involves pre-training the model on a large generic dataset and then fine-tuning it on a smaller, more specialized storytelling dataset. This allows the machine to build on existing knowledge while adapting to storytelling nuances. Another approach is implementing reinforcement learning. Here, the machine is rewarded for producing text meeting specific storytelling criteria, encouraging improvement over time.
Personalized training improves the machine’s storytelling by exposing it to individualized feedback and coaching. This can involve human evaluators providing targeted critiques and suggestions based on the specific storytelling goals.
Additionally, the machine can learn from its own previous attempts at storytelling, refining techniques based on performance and user feedback.
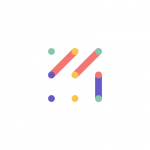
Vizologi is a revolutionary AI-generated business strategy tool that offers its users access to advanced features to create and refine start-up ideas quickly.
It generates limitless business ideas, gains insights on markets and competitors, and automates business plan creation.