Trend Analysis with ANOVA: Breaking It Down for Beginners
Are you new to trend analysis and ANOVA? Or do you need a refresher on the basics? Look no further! In this article, we will explain the trend analysis process with ANOVA in an easy-to-understand way for beginners.
By the end, you will better grasp how to use this statistical technique to analyze trends and make informed decisions. Let’s dive in and uncover the basics of trend analysis with ANOVA!
What is Trend Analysis ANOVA?
ANOVA is a tool for comparing means from different groups. It helps determine if there is a significant difference among the groups. This analysis is particularly useful for data from experiments or studies with multiple factors. The F-value in ANOVA indicates whether the group means are significantly different. A large F-value suggests significant differences, while a small F-value indicates similarity. ANOVA should be used when only two groups are needed, as a t-test can be used instead.
It’s essential to check ANOVA assumptions such as normality and homogeneity of variances to avoid inaccurate results.
Why Use ANOVA for Trend Analysis?
ANOVA is a statistical tool used to compare the means of three or more groups for significant differences.
The steps for using ANOVA in trend analysis are:
- Collecting data from multiple groups or periods
- Calculating the mean and variance for each group
- Performing the ANOVA test to identify significant differences
ANOVA results are interpreted using the p-value.
If the p-value is less than the chosen significance level, a statistically significant difference between the groups indicates a trend.
If the p-value exceeds the significance level, there’s insufficient evidence to conclude a trend.
Steps in Trend Analysis ANOVA
The steps involved in Trend Analysis ANOVA are:
- Collecting data on a dependent variable over different periods.
- Conducting ANOVA to analyze the variations in the means of the dependent variable across the periods.
- Interpreting the results to identify any significant trends.
ANOVA is used for trend analysis because it allows for comparing means across multiple groups and is particularly useful in identifying trends over time.
Some common mistakes to avoid in Trend Analysis ANOVA include:
- Not accounting for seasonality.
- The data should not be ensured to meet the assumptions of ANOVA, such as normal distribution and homogeneity of variances.
- Incorrectly interpret the results and draw conclusions based on insignificant findings.
Understanding ANOVA Results
What F-Value Means
The F-Value in trend analysis ANOVA shows the variance ratio of the group’s means to the variance within the groups. A larger F-Value means a more significant difference among the group means. To determine if a trend is significant, compare the F-Value to the critical value for significance. If the F-Value is greater than the critical value, there is evidence to reject the null hypothesis and conclude that the group means are unequal.
The F-Value is used to interpret ANOVA results by measuring variability among the group means relative to within-group variability. This helps determine real differences in the group means instead of differences due to random sampling variation.
For example, in a study comparing teaching methods’ effectiveness, a high F-Value suggests significant differences in mean test scores between the groups.
Reading the p-Value
The p-value shows the likelihood that the observed data happened by random chance in trend analysis ANOVA. You compare it to 0.05 to see if the trend is essential. If the p-value is under 0.05, the trend is statistically significant, and you can reject the null hypothesis. If the p-value exceeds 0.05, there’s insufficient evidence to reject the null hypothesis, and the trend isn’t statistically meaningful.
Different p-values in trend analysis ANOVA can significantly impact how the data is understood and decisions are made. A smaller p-value means strong evidence against the null hypothesis. In contrast, a more significant p-value suggests weaker evidence, affecting how confident you can be in the trend’s importance or not.
How to Tell if Trends are Significant
The F-value in trend analysis ANOVA shows the variance ratio between group means to the variance within the groups.
A high F-value means the group means are more different from each other than the differences within the groups.
The p-value helps determine if trends are significant by showing the probability of obtaining the observed data if the null hypothesis were true.
A small p-value (usually less than 0.05) suggests statistically significant results, not due to random chance.
When performing trend analysis ANOVA, it’s important to check the test’s assumptions, such as normality and homogeneity of variances.
Misinterpreting results without considering the data’s context and being careful of multiple comparisons are common mistakes to avoid.
Conducting many tests can raise the chance of finding false positives.
Software Tools for Trend Analysis ANOVA
Examples of Software
Software tools for trend analysis ANOVA include statistical software packages like R, Python, and SAS. Spreadsheet applications like Microsoft Excel and Google Sheets are also commonly used.
These tools help with data visualization, hypothesis testing, and regression analysis for trend analysis ANOVA.
Trend analysis ANOVA is used in finance, marketing, healthcare, and manufacturing. It helps to identify patterns and relationships within data sets, make informed decisions based on statistical significance, and predict future outcomes.
Common Mistakes in Trend Analysis ANOVA
Ignoring Assumptions
Assumptions in trend analysis ANOVA include:
- Normal distribution of residuals
- Homogeneity of variances
- Independence of observations
Ignoring these assumptions can lead to incorrect conclusions and unreliable results in the analysis. For example, if the assumption of homogeneity of variances is not met, the ANOVA results may not be valid and could lead to incorrect inferences about the differences between groups.
This can have severe consequences for decision-making processes based on the analysis. Failure to consider these assumptions can result in flawed insights and decisions, leading to wasted resources and missed opportunities for improvement.
It is essential not to ignore these assumptions because they serve as the foundation for the validity and reliability of the ANOVA results. By ensuring that these assumptions are met, analysts can have confidence in the accuracy of their findings and the reliability of their conclusions.
Misinterpreting Results
Results from trend analysis ANOVA can be misunderstood if analysts don’t consider outliers or non-normal data. Mistakes include not transforming the data to be normal and not considering influential data points. Not fixing these mistakes can lead to wrong conclusions about trends and could affect strategic decisions. For instance, not dealing with outliers in sales data before doing trend analysis ANOVA can lead to wrong conclusions about marketing effectiveness or outside factors in sales.
These mistakes can impact the reliability of trend analysis and business decisions.
Overlooking Data Issues
Common data issues in trend analysis ANOVA include:
- Outliers
- Missing data
- Inaccurate data entry
These issues can affect the accuracy and reliability of the analysis, leading to incorrect conclusions and decisions.
To address these issues:
- Thoroughly examine the dataset for inconsistencies
- Conduct data cleaning procedures
- Impute missing data using appropriate methods
Overlooking data issues can lead to misleading insights and erroneous conclusions. This can ultimately result in ineffective strategies and poor decision-making.
Prioritizing data quality and accuracy is essential for reliable and valid trend analysis ANOVA results.
Applying ANOVA in Different Fields
In Medicine
Trend Analysis, or ANOVA, is a statistical technique used in medicine. It analyzes trends and variations in data sets. This helps identify significant changes over time and compare multiple groups or treatments.
The method is especially useful in medical research studies and clinical trials. It determines the effectiveness of different treatments or interventions.
Using ANOVA for trend analysis, researchers can identify patterns and fluctuations in patient outcomes, population health trends, and the impact of new medical protocols.
In medicine, significant trends are identified through the comparison of p-values. These help determine the probability of obtaining the observed results by chance.
If the p-value is less than a pre-determined significance level, the trend is considered statistically significant in the medical context.
This approach can help healthcare professionals make evidence-based decisions and improve patient care outcomes.
In Business
Trend Analysis, or ANOVA, is a statistical technique for determining whether there are significant differences between the means of two or more groups.
It can handle multiple groups and identify patterns and variations in the data.
Trend Analysis ANOVA involves collecting data, determining the significance level, calculating the F-value, and interpreting the P-value.
The F-Value measures the differences between the group means and indicates the significance of the trends.
The p-Value determines the probability of obtaining results by chance. Significant trends are identified when the p-Value is less than 0.05.
Common mistakes in Trend Analysis ANOVA include not checking for assumptions, misinterpreting results, and using the wrong ANOVA model.
Some software tools for Trend Analysis ANOVA include Python, R, and MATLAB.
ANOVA can be applied in different fields, such as healthcare, education, and economics.
Beginners in Trend Analysis ANOVA should focus on understanding the assumptions, practicing with sample data, and seeking guidance from experienced professionals.
In Agriculture
ANOVA is a statistical method. It’s used in agriculture to compare the means of more than two data groups. For example, it can compare crop yields in different field conditions.
The F-value in ANOVA tells us whether the differences between the means are likely due to actual effects or just random variation.
The F-value can help determine if factors like soil quality or irrigation methods significantly impact crop production in agriculture.
To understand if trends are significant in agriculture, researchers look at the p-value associated with the F-value.
A small p-value suggests that the observed trends are unlikely to result from random chance. This indicates that the factors being analyzed have a real impact on agricultural outcomes.
For instance, a low p-value when analyzing the impact of different types of pesticides on crop pest control would suggest that the pesticide used significantly affects the pest population.
Tips for Beginners in Trend Analysis ANOVA
Start with Clear Goals
When conducting trend analysis ANOVA, gather historical data and identify the time periods to be analyzed. Then, organize the data into groups based on specific criteria. After that, ANOVA tests will be run to compare the means across these groups.
ANOVA should be used for trend analysis because it allows for the comparison of multiple groups simultaneously, providing valuable insights into any variations or patterns in the data over time. Common mistakes to avoid in trend analysis ANOVA include misinterpreting the results due to insufficient data or using inappropriate criteria to group the data.
Additionally, failing to account for seasonal variations or to validate ANOVA’s assumptions can lead to inaccurate conclusions. By starting with clear goals for trend analysis ANOVA, researchers can ensure that the process is focused and the results are reliable.
Get Comfortable with Data
Trend Analysis ANOVA is a statistical technique that compares means from different groups to find significant differences. It helps analyze trends by comparing more than two groups at once, helping to identify patterns. The F-value in ANOVA represents the variability ratio between group means and within-group variability. A larger F-value indicates a tremendous difference between group means, suggesting a significant trend or pattern.
For example, in a study comparing students’ test scores by grade level, ANOVA could determine if there’s a significant performance difference. This information is valuable for data-driven education, business, or healthcare decisions.
Practice Interpreting ANOVA Output
Trend analysis ANOVA is a method for analyzing data and checking for significant differences between the means of three or more groups. It’s commonly used in research to study the impact of an independent variable on a dependent variable across various levels, like time or treatment groups.
The F-Value in ANOVA tells us if the means of the groups are significantly different. Meanwhile, the P-Value shows the likelihood of getting the observed results by chance, with a lower p-value indicating less chance of random variation.
When doing trend analysis ANOVA, it’s crucial to avoid common mistakes like not checking for normality or homogeneity of variances and not confirming that the data meets the assumptions of ANOVA. Also, failing to follow up significant ANOVA results with post-hoc tests can lead to inappropriate conclusions.
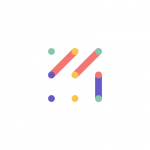
Vizologi is a revolutionary AI-generated business strategy tool that offers its users access to advanced features to create and refine start-up ideas quickly.
It generates limitless business ideas, gains insights on markets and competitors, and automates business plan creation.