What is an AI language model? Explained!
Have you ever considered how your smartphone predicts the next word you’ll type? Or how do virtual assistants like Siri or Alexa understand and respond to your questions? The answer is AI language models. These systems have changed the way technology understands and processes human language. They power search engines and generate text, transforming how we interact with technology. Let’s explore what an AI language model is and how it works.
Understanding AI Language Models
AI language models come in two main types: statistical and neural. Statistical models use probabilities to predict word sequences, while neural models, like Recurrent Neural Networks (RNNs) and transformers, aim to improve context capture and address the limitations of statistical models.
The engine behind AI language models, such as GPT-3, relies on machine learning algorithms trained to predict the next word in a sentence based on context. For instance, GPT-3 uses a large dataset to generate human-like text, while ChatGPT is fine-tuned for conversations.
These language models have diverse applications, including content generation, question answering, sentiment analysis, and machine translation. However, they have limitations in tasks requiring reasoning and general intelligence despite their ability to generate human-like text.
How Do AI Language Models Work?
AI language models work by predicting word sequences in sentences. They are trained to understand and generate human-like text. This has applications in content generation, part-of-speech tagging, question answering, text summarization, sentiment analysis, conversational AI, machine translation, and code completion.
The primary components and processes involve predicting the next word in a sentence based on context. Recurrent Neural Networks and transformers contribute to AI language models by addressing the limitations of statistical models and improving context capture.
Potential advancements for AI language models include further improvements in understanding and generating human-like text, as well as ethical considerations surrounding their development and use.
Different Types of AI Language Models
Simple Language Models
Simple language models in AI use statistics and probabilities to predict word sequences. They are important in natural language processing and understanding. These models focus on basic predictions and language understanding. They are used in content generation, part-of-speech tagging, question answering, text summarization, sentiment analysis, conversational AI, machine translation, and code completion.
They are used in various industries to generate content, analyze sentiments, and provide automated responses. However, simple language models have limitations in handling reasoning and general intelligence tasks. Advanced models like GPT-3 by OpenAI have been developed to tackle more complex language and reasoning tasks in AI.
Advanced Language Models
Advanced language models are better at understanding and generating complex text than simple models. Simple models can only predict the next word based on previous ones, while advanced models like GPT-3 can handle more sophisticated contexts.
Cutting-edge transformers, an advanced language model, improve context capture compared to traditional recurrent neural networks. This allows them to understand surrounding words better and generate more cohesive and relevant text.
Future developments in AI language models include refining natural language processing tasks like content generation, question answering, and sentiment analysis. Ethical concerns about bias and fairness are also important. Moreover, there’s potential for more sophisticated conversational AI and enhanced machine translation capabilities.
The Engine Behind AI-Language Models: RNNs and Transformers
Looping Patterns with Recurrent Neural Networks
Recurrent Neural Networks create looping patterns in AI language models. They use feedback loops to capture and analyze sequential data, making them effective for modeling language structures.
For example, in language processing tasks, RNNs can predict the next word in a sentence based on the context of previous words, generating looping patterns.
RNNs excel in text generation, speech recognition, and machine translation. They enhance the efficiency and performance of AI language models by improving context capture and understanding long-range dependencies in the text. This helps address the limitations of statistical models and provides more accurate predictions in language-related tasks.
Cutting-Edge Transformers
Cutting-edge Transformers in AI language models are a big step forward in technology for processing human language. Models like GPT-3 and ChatGPT use neural networks to understand complex language patterns and produce text very close to human writing. Unlike older models, these cutting-edge Transformers are much better at creating content, answering questions, analyzing feelings, and finishing code.
Their ability to understand and create human-like text has many possible uses in healthcare, education, customer service, and finance. In these fields, cutting-edge Transformers can help make reports automatically, personalized teaching, and chatbot conversations, improving things and giving users a better experience. We can see the impact of these models in making more advanced AI systems for talking, tools to translate languages, and ways to make content shorter.
Overall, the arrival of cutting-edge transformers in AI language models has created remarkable progress and opportunities for new ideas in many fields.
Influential Ways AI Language Models Help Us
Things AI Language Models Can Do
AI language models can do many things. They can generate content, tag parts of speech, answer questions, summarize text, analyze sentiment, have conversations, translate languages, and complete code. They help in different industries by understanding and creating human-like text. But, they have limits in tasks that need reasoning and general intelligence. Right now, they can’t fully copy human cognitive abilities.
These limits affect their use in areas needing higher-level thinking and understanding. But AI models keep getting better, and they can help improve different fields and industries in the future.
Things AI Language Models Can’t Do
AI language models can understand and generate human-like text, but they have limitations. They struggle with reasoning and general intelligence.
For example, they have difficulty detecting and addressing bias or sensitive language. This can lead to outputs that reinforce stereotypes or use unacceptable language. Also, these models can’t accurately interpret complex or ambiguous language, which can lead to misunderstandings and incorrect interpretations. Understanding context and nuance in language is also challenging for AI language models. This makes it difficult for them to produce accurate and meaningful content or address specific communication needs. Despite their advancements, AI language models still face significant barriers in comprehending and responding appropriately to the intricacies of human language. This makes them unsuitable for tasks requiring high levels of understanding, interpretation, and unbiased representation.
Big Names in AI Language Models and What They Do
Chatting with GPT-3
GPT-3, a powerful language model, has many uses in chatting and conversation tasks. It’s great at generating content, answering questions, summarizing text, analyzing sentiment, translating languages, and completing code.
For conversations, there’s ChatGPT, which is fine-tuned specifically for this purpose. It can understand and generate human-like text, making it a valuable tool for chatting and talking to users.
Compared to other AI language models, like statistical models and neural language models, GPT-3 stands out for its conversational abilities. It uses advanced techniques and vast amounts of data to generate contextually relevant and coherent responses. While it may have limitations in tasks involving reasoning and general intelligence, its chatting and conversation skills are impressive and make a big contribution to the field of natural language processing.
Google’s BERT at Work
Google’s BERT is an important advancement in AI language models. It focuses on understanding the context of words in search queries. Unlike other models, BERT analyzes the full context of a word by looking at the words before and after it. This helps BERT better understand the subtleties of language and give more accurate search results. BERT is currently used in various applications, such as improving search engine results and enhancing natural language processing tasks.
In the future, it’s expected to play a key role in advancing AI language models, especially in tasks related to understanding and generating human-like text.
Nvidia and Microsoft’s Team-Up with MT-NLG
Nvidia and Microsoft teaming up with MT-NLG in the field of AI language models is a big deal. This collaboration could significantly impact how advanced language models are developed and used. These companies could create more powerful and versatile language models that work across different industries. This partnership might lead to breakthroughs in language modeling technology, making AI systems better at understanding and using human language.
The future looks bright for AI language models because of this joint venture.
Google’s Chatty LaMDA
Google’s Chatty LaMDA is different from other AI language models. It can have better conversations and understand context more effectively. It focuses on making conversations more natural and is specifically trained for chat-based tasks. Chatty LaMDA can be used in real-life situations like creating content, answering questions, summarizing texts, and developing chat-based AI, making human-machine interactions smoother.
Looking Ahead: The Road for AI Language Models
As AI language models continue to advance, they will likely face challenges related to ethical considerations, limitations in reasoning and general intelligence, and the need for continued improvement in context capture. Despite these obstacles, AI language models have the potential to evolve and improve in the coming years through the development of more advanced neural network architectures and ongoing training with vast and diverse datasets.
Additionally, as technology progresses, AI language models can be adapted and applied to new industries and fields, such as healthcare, finance, and education, to enhance content generation, conversational AI, code completion, and more. By expanding their applications and refining their capabilities, AI language models have the potential to play a critical role in a wide range of industries and sectors in the future.
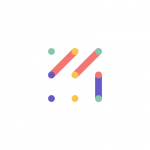
Vizologi is a revolutionary AI-generated business strategy tool that offers its users access to advanced features to create and refine start-up ideas quickly.
It generates limitless business ideas, gains insights on markets and competitors, and automates business plan creation.