What’s a Risk Assessment Algorithm? Here’s the Simple Answer!
Curious about what a risk assessment algorithm is and how it works? You’re not alone.
Understanding this concept is simpler than you might think. A risk assessment algorithm is a tool that helps organizations evaluate potential risks and make informed decisions.
By using data and analysis, this algorithm helps identify potential threats and their likelihood of occurring.
In this article, we’ll break down the basics of risk assessment algorithms and why they matter. Get ready to demystify this important tool!
Understanding Risk Assessment Algorithms
What is a Risk Assessment Algorithm?
Some potential improvements to risk assessment algorithms are:
- Preserve human oversight and careful discretion when implementing machine learning algorithms.
- Ensure algorithm transparency.
- Examine the data for potential harm to any group.
- Build next-generation risk algorithms that predict reductions in risk caused by supportive interventions.
Bias in algorithms can be addressed by:
- Carefully selecting outcomes that reflect true underlying crime rates.
- Examining the data for potential harm to any group.
- Ensuring that any difference in impacts by race is taken into consideration.
Ethical considerations in developing and using risk assessment algorithms include:
- Ensuring transparency and interpretability in the algorithms to facilitate scrutiny of the accuracy of all information.
- Addressing potential biases to reduce racial disparity in sentencing.
- Making the factors that heavily influence the score explicit to the courts to allow for an explicit discussion about the mitigating or aggravating impact of youth at sentencing.
How Do These Algorithms Work?
Risk assessment algorithms determine an individual’s level of risk by using special software. The software analyzes the answers from a questionnaire completed by the defendant when booked into the criminal justice system.
These algorithms generate predictive scores like “risk of recidivism” and “risk of violent recidivism” based on the data analysis. They utilize various factors such as age, prior criminal history, and personal background to calculate risk scores.
It’s important for the algorithms to consider factors that reflect true underlying crime rates to avoid perpetuating biases in the criminal justice system.
Yet, these algorithms can have limitations and biases, including racial and age biases. There’s also the potential for them to contribute to racial disparity in sentencing and obscure certain factors, like age, leading to inappropriate sentences.
Where Are Risk Assessment Algorithms Used?
Risk assessment algorithms are commonly used in the criminal justice system. They predict future criminal behavior and recommend sentence length and severity for each defendant.
The COMPAS algorithm is an example of this. It has been successful at federal and state levels in the US, but it has also raised concerns about reliability and bias, including racial and age biases.
The opaque nature of these algorithms raises legal and ethical concerns about their accuracy and potential to worsen biases. Validity and training issues have also been disregarded, pointing to challenges in this area.
Problems with Risk Assessment Algorithms
Can Algorithms be Unfair?
Algorithms can be unfair. They may perpetuate biases in the criminal justice system, like the COMPAS risk assessment algorithm, which was claimed to be biased against Black individuals compared to white individuals. The opaqueness of these algorithms raises legal and ethical concerns, particularly regarding risks for different groups of people.
Improperly validated risk assessments can contribute to racial disparity in sentencing, as the data often reflects a system where racial identity affects arrest probability. Mistakes made by algorithms, such as improperly weighing certain factors, can lead to unfair and inappropriate sentencing, potentially perpetuating existing biases in the criminal justice system. The lack of transparency and oversight in these algorithms makes it difficult for judges and parole authorities to understand and mitigate the impact of certain factors, ultimately influencing criminal justice outcomes for different groups of people.
Risks for Different Groups of People
Many risk assessment algorithms often give higher risk scores to young people. This especially affects racial minority groups. Algorithms can mix up being young and being responsible, which might lead to biases in court decisions.
Algorithms often make mistakes by not considering a person’s mitigating factors and higher liability because of their age. They also fail to address potential biases.
Lastly, there’s not enough transparency about how age affects risk assessment tools. This makes it hard to think about youthfulness as a mitigating factor in risk assessment algorithms.
Mistakes Made by Algorithms
Common mistakes made by risk assessment algorithms:
- Failure to disclose detailed information on how the risk score was calculated
- Perpetuating existing biases in the criminal justice system
- Exacerbating biases
- Negative impact on different groups of people, especially in terms of racial disparity in sentencing
- Aggravating effect of youth on the risk score
To improve algorithm accuracy:
- Preserve human oversight and careful discretion in implementing machine learning algorithms
- Ensure algorithm transparency
- Examine data for potential harm to any group
- Build next-generation risk algorithms predicting reductions in risk due to supportive interventions.
Real Stories of Risk Assessment
What Happened in Two Cases of Shoplifting?
In two cases, shoplifting occurred. Both cases involved non-violent acts of theft. The first case involved a young white woman trying to steal makeup from a drug store. The store manager called authorities. The second case involved a black man trying to steal shoes and being detained by store security.
Two cases of drug possession also occurred, neither involving violence. In the first case, a white person was caught with a small amount of marijuana and received a fine and probation, avoiding conviction through an arrangement with a public attorney. In the second case, a black person was caught dealing cocaine and was promptly arrested, receiving a mandatory prison sentence upon arraignment.
In risk assessment algorithms, there’s a notable difference in risk scores for black and white people. Black individuals often receive higher scores for similar non-violent offenses. This can lead to harsher sentences for black people due to the increased perception of risk by judges and parole officers.
What Happened in Two Cases of Drug Possession?
Two drug possession cases used risk assessment algorithms for sentencing. In one case, the defendant got a six-year sentence based on their COMPAS score, a predictive tool in the criminal justice system. The Wisconsin Supreme Court also backed using automated programs for sentencing. Both cases raise concerns about bias and data accuracy. Despite the controversy, these algorithms are widely used in federal and state levels, highlighting their relevance in the criminal justice system.
Transparency and interpretability in risk score calculations are crucial for judicial decisions’ impact.
How Risk Scores Differ for Black and White People
Risk scores are different for black and white people based on their race. The COMPAS algorithm performed worse for Black individuals than for white individuals on one specific measure. These differences are influenced by statistical flaws, perpetuating and exacerbating biases in the criminal justice system. The impact of these differences can result in enhanced risk while diminishing blameworthiness, leading individuals to be labeled with condemning labels.
These differences in risk scores for different racial groups have been recognized as a pivotal issue by the National Institute of Justice. This has sparked concerns about the validity and bias of risk assessment computer models, leading to limited research on their real-world use.
When the Algorithm Gets it Wrong
Risk assessment algorithms can make mistakes by not considering certain factors, like age, when calculating risk scores. This can lead to inflated sentences. These mistakes can amplify biases in the criminal justice system and contribute to racial disparities in sentencing. Factors contributing to these mistakes include lack of transparency in the algorithm’s calculation. This makes it hard for defendants or legal professionals to check the accuracy of information presented at sentencing.
Improper validation of risk assessments can also perpetuate racial disparity and worsen biases in the criminal justice system.
Making Risk Assessments Better
Steps to Improve Algorithms
To improve algorithms:
- Prioritize transparency and interpretability to make algorithms more fair and unbiased.
- Carefully examine the data input to avoid potential harm to any group.
- Preserve human oversight when implementing machine learning algorithms to reduce biases.
- Select outcomes reflecting true underlying crime rates to reduce risks for different groups.
- Examine the real-world impacts of risk assessment algorithms, including potential differences based on race.
- Build next-generation risk algorithms that predict decreases in risk due to supportive interventions to minimize potential risks for different groups of individuals.
- Guarantee algorithm transparency to minimize errors in risk assessment.
- Ensure input factors can be understood by all involved parties.
- Address and consider conflicting roles of factors such as age in risk assessment tools to reduce errors in how scores are interpreted.
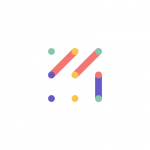
Vizologi is a revolutionary AI-generated business strategy tool that offers its users access to advanced features to create and refine start-up ideas quickly.
It generates limitless business ideas, gains insights on markets and competitors, and automates business plan creation.