Driving Innovation in Financial Services
In recent years, the financial sector has witnessed a significant transformation driven by advancements in technology and data analytics. Data science has emerged as a critical component in enhancing financial services, enabling institutions to make informed decisions, mitigate risks, and improve customer experiences. Data science development firm play a pivotal role in this evolution by providing the expertise and technological solutions necessary to harness the power of data. This article explores key applications of data science in finance, including fraud detection, risk management, and algorithmic trading.
The Role of Data Science in Finance
A. Definition and Scope
Data science is an interdisciplinary field that uses scientific methods, algorithms, and systems to extract knowledge and insights from structured and unstructured data. Data science has many applications in the financial industry, ranging from predicting market trends to analyzing transaction patterns. Financial institutions can improve their operations and provide better services to their clients by utilizing the massive amounts of data generated on a daily basis.
B. Data Science Development Companies Are Important
Customized solutions that assist financial institutions in navigating the challenges of data analysis are the speciality of data science development companies. These companies assemble groups of engineers, analysts, and data scientists who collaborate with financial institutions to create cutting-edge tools and tactics. Successful partnerships between financial institutions and data science companies have led to increased operational effectiveness, better risk management procedures, and more successful client engagement tactics.
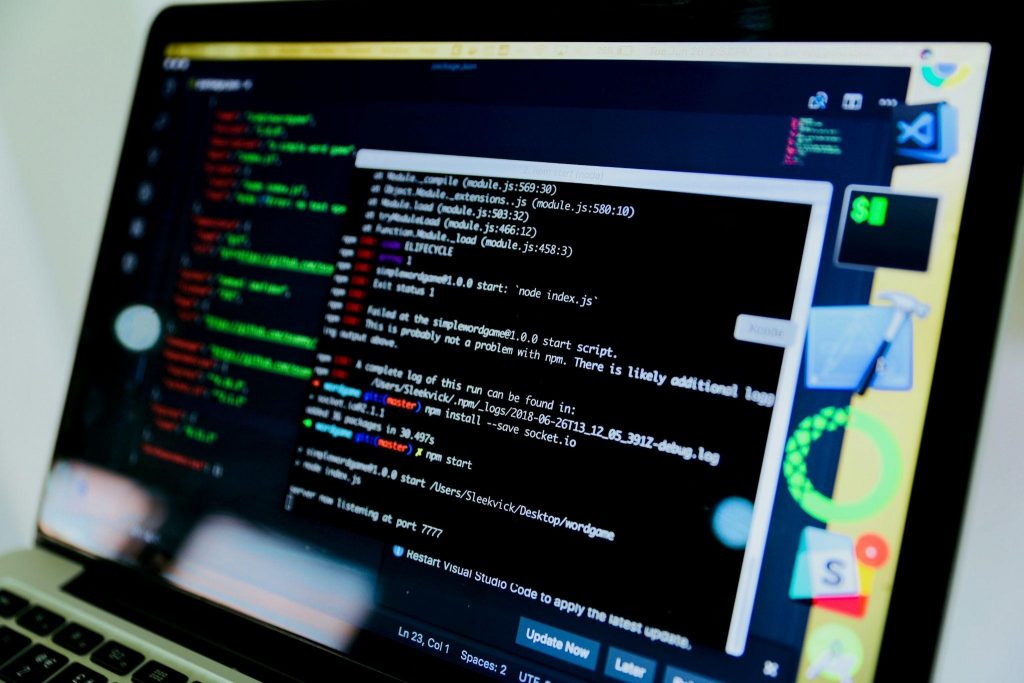
Applications of Data Science in Finance
A. Fraud Detection
- Machine Learning Techniques
Machine learning algorithms are at the forefront of fraud detection efforts in finance. By analyzing historical transaction data, these algorithms can identify patterns indicative of fraudulent activities. Supervised learning models are trained on labeled datasets to recognize known fraud cases, while unsupervised learning models detect anomalies without prior knowledge of fraudulent behaviour. - Anomaly Detection
Anomaly detection methods play a crucial role in identifying unusual patterns that may signify fraud. Techniques such as clustering and statistical analysis help flag transactions that deviate significantly from typical behavior. Case studies have shown that financial institutions employing these methods have successfully prevented substantial losses through early detection. - Real-Time Analytics
The ability to process data in real-time is essential for effective fraud detection. Advanced technologies such as big data analytics and cloud computing enable financial institutions to monitor transactions continuously, allowing for immediate intervention when suspicious activities are detected.
B. Risk Management
- Risk Assessment Models
Data science is instrumental in developing comprehensive risk assessment models that evaluate various types of financial risks—market risk, credit risk, and operational risk. Predictive analytics allows organizations to forecast potential risks based on historical data and market conditions. - Scenario Analysis and Stress Testing
Financial institutions utilize scenario analysis and stress testing to evaluate their exposure to different market conditions. By simulating various economic scenarios, data science helps organizations understand potential vulnerabilities and develop strategies for risk mitigation. - Regulatory Compliance
Ensuring compliance with regulatory requirements is a significant challenge for financial institutions. Data science aids in monitoring compliance risks by providing tools for analyzing transaction data against regulatory standards, thus reducing the likelihood of violations.
C. Algorithmic Trading
- Automated Trading Systems
Algorithmic trading relies heavily on data science to execute trades based on predefined criteria without human intervention. By analyzing vast datasets in real-time, these systems can capitalize on market opportunities quickly and efficiently. - High-Frequency Trading (HFT)
High-frequency trading strategies utilize sophisticated algorithms to execute a large number of orders at extremely high speeds. Data science plays a crucial role in developing these algorithms, enabling traders to respond rapidly to market changes. - Market Analysis and Sentiment Analysis
Data science techniques are also employed to analyze market trends and investor sentiment, which can inform trading strategies. By processing news articles, social media posts, and other textual data sources, firms can gauge public sentiment and adjust their trading approaches accordingly.
Challenges Faced by Data Science Development Firms in Finance
A. Data Quality and Integration
One of the primary challenges faced by data science development firms is ensuring high-quality data from multiple sources. Inconsistent or incomplete datasets can lead to inaccurate analyses and flawed decision-making processes.
B. Regulatory Challenges
Navigating the complex regulatory landscape is another hurdle for data science firms working with financial institutions. Compliance with ever-evolving regulations requires continuous monitoring and adaptation of analytical models.
C. Ethical Considerations
As with any technology reliant on data analysis, ethical considerations surrounding privacy and bias must be addressed proactively. Ensuring transparency in algorithmic decision-making is essential for maintaining trust among clients and stakeholders.
Future Trends in Data Science for Financial Services
A. Advancements in AI and Machine Learning
The future of data science in finance will be shaped by advancements in artificial intelligence (AI) and machine learning technologies. As these technologies continue to evolve, they will enable more sophisticated analyses and predictive capabilities.
B. Increased Focus on Personalization
Financial services are increasingly moving towards personalization driven by data analytics. Tailoring products and services based on individual customer preferences will become a key differentiator for financial institutions.
C. The Rise of FinTech Companies
FinTech companies are leveraging data science to disrupt traditional financial services by offering innovative solutions that enhance customer experiences while streamlining operations.
Conclusion
Data science development firms are at the forefront of driving innovation within the financial services sector by harnessing the power of data analytics to improve fraud detection, risk management, and algorithmic trading practices. As financial institutions increasingly embrace these technologies, they stand to gain significant advantages in efficiency, compliance, and customer engagement strategies. To remain competitive in this rapidly evolving landscape, organizations must continue investing in data-driven solutions that enhance their operational capabilities.
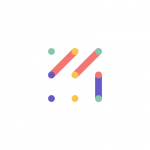
Vizologi is a revolutionary AI-generated business strategy tool that offers its users access to advanced features to create and refine start-up ideas quickly.
It generates limitless business ideas, gains insights on markets and competitors, and automates business plan creation.