GPT Magic: Is It Part of the Generative AI Family?
Have you ever heard of GPT magic? It’s the latest technology creating waves in the world of Generative AI. But is it truly part of the Generative AI family? Let’s dive into the fascinating world of GPT magic and explore its connection to the broader realm of Generative AI.
What is Generative AI?
The Pieces of the Puzzle: How AI is Put Together
Generative AI and GPT models are transforming various industries and job functions with their ability to create diverse content, such as music, art, and virtual environments. The GPT models operate on neural network-based language prediction models built on the Transformer architecture. Specifically, transformer neural networks utilize encoder and decoder modules to process input sequences and generate accurate predictions.
1.Encoder:
- The transformer’s encoder block separates words into embeddings and assigns weight to each, capturing contextual information from an input sequence.
- Position encoders differentiate semantic meanings and prevent ambiguous interpretations of words in sentences.
2.Decoder:
- The decoder uses the vector representation from the encoder to predict the requested output, with built-in self-attention mechanisms to focus on different parts of the input.
Generative AI models like ChatGPT and DALL-E showcase extensive capabilities and applications. However, their influence also raises concerns about producing inaccurate or inappropriate content. Nonetheless, the impact of generative AI continues to unfold, presenting opportunities for revolutionizing job functions and industries.
Understanding the technical underpinnings of GPT models and their potential impact on various fields is crucial in this transformative computing era. For more insights into generative AI models and their practical applications, connecting with experts in the field can be valuable.
Types of Generative AI: GPT and Friends
What CEOs Need to Know About AI Wonders
AI Wonders for CEOs
When considering AI GPT models, it’s important for CEOs to understand that these generative AI systems, a subset of machine learning, enable computers to create diverse content such as music, art, and virtual environments. For example, generative AI models like ChatGPT and DALL-E have practical applications in product design and business optimization, showing the potential to revolutionize various job functions. However, it’s crucial to recognize that while they have shown positive impacts, the full scope and risks of their influence remain unknown.
Furthermore, CEOs should be aware that the development of these generative AI models is a significant undertaking, primarily by tech giants like OpenAI, Meta, and DeepMind because of the extensive resources and expertise required. These models have the potential to reshape various industries, even as their impact continues to unfold.
In addition, understanding various generative AI models and their histories, OpenAI vs. Azure OpenAI, ChatGPT, and how to get started working with these tools is crucial in this revolutionary computing era. For more insights, connect with Francesca Lazzeri, Ph.D., on LinkedIn.
AI vs. Machine Learning: What’s the Big Idea?
Different Learning for Machines: The Main Models
Neural Networks: These are the foundation of generative AI, particularly the GPT models. For example, there are transformer and convolutional neural networks, each with distinct features and applications. The transformer neural network, the architecture used by GPT models, employs self-attention mechanisms to enhance natural language processing tasks.
Encoder: The transformer network utilizes an encoder component to process text inputs as mathematical embeddings, capturing contextual information from an input sequence. This allows the GPT models to understand the relevance of words in a sentence and differentiate between semantic differences in language.
Decoder: After processing input from the encoder, the decoder module predicts the requested output using self-attention mechanisms and complex mathematical techniques. Unlike its predecessors, transformers can process the entire input at once during the learning cycle, enabling them to provide fluent answers to various inputs.
Generative AI Training: Training of GPT models involves processing massive language datasets with hundreds of billions of parameters. The transformer architecture’s capacity to analyze natural language allows the GPT models to dynamically attend to different parts of the input and generate long responses, making them capable of creating content with literary styles such as Shakespeare-inspired phrases and sentences.
How Generative AI Thinks in Words: Text-Based Models
Generative AI systems, like GPT models, are capable of creating diverse content, including text-based outputs. GPT models, which are neural network-based language prediction models, have the ability to analyze natural language queries and predict responses based on their understanding of language. For instance, when prompted to generate content inspired by Shakespearean literature, a GPT model can reconstruct phrases and sentences with a similar literary style.
These models rely on large language datasets and use transformer neural network architectures, which employ self-attention mechanisms to focus on different parts of the input text while processing it. The encoder component captures contextual information from an input sequence, while the decoder uses the vector representation to predict the requested output.
Compared to their predecessors, transformers are more parallelizable and can process the entire input at once, which contributes to their proficiency in generating responses.
With their potential to revolutionize various industries, GPT models are gaining attention for their practical applications across different fields and their ability to provide fluent answers to a wide range of inputs. Their impact continues to unfold, making them a significant advancement in the field of AI.
From A to AI: Building a Generative Model Step-by-Step
Generative Models and GPT: Understanding the Key Components
Generative AI, a subset of machine learning, enables computers to create diverse content, such as music, art, and virtual environments, with practical applications in product design and business optimization. Generative AI models like ChatGPT and DALL-E have shown significant potential to revolutionize various job functions, impacting fields like AI adoption and how jobs are performed.
Generative AI models are a significant undertaking, primarily developed by tech giants like OpenAI, Meta, and DeepMind due to the extensive resources and expertise required, with both positive impacts and underlying risks as their influence continues to unfold.
Key components of generative models like ChatGPT include:
- Built on large language models like GPT-3 and GPT-4
- Fine-tuning for conversation using reinforcement learning techniques
- Can also be used for completion and conversation with entirely new base model
To use ChatGPT and GPT-4 with Azure OpenAI Service, follow the steps provided and start exploring OpenAI capabilities with a no-code approach through the Azure OpenAI Studio ChatGPT playground.
Understanding these various generative AI models’ history, their significance in this revolutionary computing era, and the relationship between OpenAI and Azure OpenAI are essential for anyone interested in AI advancement.
What Generative AI Can Make: The Many Outputs
Generative AI represents a subset of machine learning that allows computers to generate diverse content, including music, art, and virtual environments. These systems, such as ChatGPT and DALL-E, have demonstrated practical applications in product design and business optimization and have the potential to transform various job functions. While the positive impact of generative AI models is evident, the full scope and potential risks of their influence remain largely unknown.
Notably, generative AI is a complex field primarily tackled by major tech companies like OpenAI, Meta, and DeepMind due to the substantial resources and expertise required. The outputs generated by these models can range from highly realistic and useful content to inaccurate or inappropriate results. However, their potential to revolutionize various industries cannot be overlooked, even as their impact continues to evolve.
Generative AI models have made significant contributions across diverse fields and have the potential to redefine how various jobs are performed. As AI adoption continues to grow, models like ChatGPT, built on large language models like GPT-3 and GPT-4, have emerged as important tools. Azure OpenAI Service, in collaboration with Microsoft, provides access to OpenAI models through REST API calls, making it easier to leverage the capabilities of generative AI.
AI Problem Solvers: What Can Generative Models Do?
AI Problem Solvers: What Generative Models Can Achieve
Generative models like GPT, part of artificial intelligence (AI), have the ability to create diverse content, ranging from music, art, to virtual environments. For example, ChatGPT and DALL-E are at the forefront of this technology, showing promising potential in product design and business optimization. However, the full extent and potential drawbacks of their influence are still not entirely understood.
Primarily developed by tech giants like OpenAI and Meta, generative AI remains a significant and complicated undertaking due to the extensive resources and expertise required. While these models have shown positive impacts, they also have the potential to generate inaccurate or inappropriate results. Nevertheless, their transformative power across various industries is undeniable.
Generative AI models like ChatGPT have already begun reshaping how jobs are performed. In the last five years, AI adoption has more than doubled, demonstrating the increasing importance of this technology. With tools like Azure OpenAI and concepts like GPT-3, Codex, and DALL·E, generative AI models are becoming more accessible and have shown promising applications in various fields.
AI’s Challenges: The Limits and How We Can Push Them
Generative AI and its Challenges: Pushing the Limits
Generative AI, such as ChatGPT and DALL-E, is a subcategory of machine learning, allowing computers to produce diverse content like music, art, and virtual environments. These models have contributed to product design, business optimization, and have the potential to reshape various job functions. While they present numerous opportunities, the full scope and risks of their influence remain uncertain.
Generative AI is primarily developed by tech giants such as OpenAI and Meta due to the extensive resources and expertise required. The models’ outputs can range from highly realistic and useful content to inaccurate or inappropriate results, posing both significant opportunities and challenges.
These models could revolutionize various industries, yet the complete impact is still unfolding and requires careful navigation. The key challenges of generative AI lie in ensuring the responsible and ethical use of these capabilities to maximize their potential benefits and minimize potential risks as the technology continues to evolve.
- Generative AI enables computers to create diverse content such as music, art, and virtual environments.
- Tech giants like OpenAI and Meta are leading the development of generative AI due to the extensive resources and expertise required.
- The output of generative AI models varies widely, from highly realistic and useful content to inaccurate or inappropriate results.
- The full scope and impact of generative AI on various industries is still unfolding and requires responsible and ethical use to maximize benefits and minimize risks.
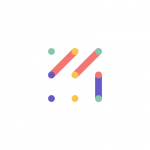
Vizologi is a revolutionary AI-generated business strategy tool that offers its users access to advanced features to create and refine start-up ideas quickly.
It generates limitless business ideas, gains insights on markets and competitors, and automates business plan creation.